
Joe Marshall — Gemini experiment
@2025-07-11 14:59 · 5 hours agoJoe Marshall — An observation
@2025-07-10 23:53 · 20 hours agoif err != nil
as “inshallah”.
vindarel — Lisp error handling (advanced): how handler-bind doesn't unwind the stack
@2025-07-08 09:45 · 3 days agoI updated the Condition Handling page on the Common Lisp Cookbook to show what it means that handler-bind
“doesn’t unwind the stack”, along with a couple real-world use cases.
This time I must thank Ari. I originally wrote the page (with
contributions from jsjolen, rprimus and phoe), starting from Timmy
Jose “z0ltan”’s article (linked in the introduction) and the books I
had at my disposal. These don’t show handler-bind
’s power and
use-cases like this, focusing on restarts (which this page certainly
could explain better, it shows they aren’t part of my daily
toolbelt). So I learned about the real goodness by chance while
reading @shinmera’s code and, shame on me, I didn’t update the
Cookbook. My video course has been well and complete for years though
;) I needed fresh eyes and that happened with Ari. He asked if I
offered 1-1 video lisp mentoring. I didn’t, but now I do!. So, as
we talked about a million things (lisp and software development in
general) and eventually worked on condition handling, I realized this
page was lacking, and I took an extra 1h 40min to update it,
“backporting” content from my video course.
These days my attention is more turned towards my tutorials for web development in Common Lisp, my CL projects and libraries, and less to the Cookbook alone like before, but for this edit to the Cookbook 2 things were new and important:
- I had fresh eyes again, thanks to the lisp mentoring
- I had an extra motivation to do the edit in the context of the 1-1 session: I felt it’s part of the job. By the way, we settled on 40USD an hour and I have a couple more slots available in the week ;) Please contact me by email (@ vindarel (. mailz org)) Thanks! [/end of self plug].
So what is this all about? handler-bind
, unlike handler-case
,
doesn’t unwind the stack: it shows us the full backtrace and gives us
absolute control over conditions and restarts.
It’s particularly necessary if you want to print a meaningful
backtrace. We’ll give another development tip, where you can decide to either
print a backtrace (production mode) or accept to be dropped into the
debugger (invoke-debugger
).
Feel free to leave feedback, in the comments or in the Cookbook issues or PR.
Table of Contents
Absolute control over conditions and restarts: handler-bind
handler-bind is what to use when we need absolute control over what happens when a condition is signaled. It doesn’t unwind the stack, which we illustrate in the next section. It allows us to use the debugger and restarts, either interactively or programmatically.
Its general form is:
(handler-bind ((a-condition #'function-to-handle-it)
(another-one #'another-function))
(code that can...)
(...error out...)
(... with an implicit PROGN))
For example:
(defun handler-bind-example ()
(handler-bind
((error (lambda (c)
(format t "we handle this condition: ~a" c)
;; Try without this return-from: the error bubbles up
;; up to the interactive debugger.
(return-from handler-bind-example))))
(format t "starting example...~&")
(error "oh no")))
You’ll notice that its syntax is “in reverse” compared to
handler-case
: we have the bindings first, the forms (in an implicit
progn) next.
If the handler returns normally (it declines to handle the condition), the condition continues to bubble up, searching for another handler, and it will find the interactive debugger.
This is another difference from handler-case
: if our handler
function didn’t explicitely return from its calling function with
return-from handler-bind-example
, the error would continue to bubble
up, and we would get the interactive debugger.
This behaviour is particularly useful when your program signaled a simple condition. A simple condition isn’t an error (see our “conditions hierarchy” below) so it won’t trigger the debugger. You can do something to handle the condition (it’s a signal for something occuring in your application), and let the program continue.
If some library doesn’t handle all conditions and lets some bubble out
to us, we can see the restarts (established by restart-case
)
anywhere deep in the stack, including restarts established by other
libraries that this library called.
handler-bind doesn’t unwind the stack
With handler-bind
, we can see the full stack trace, with every
frame that was called. Once we use handler-case
, we “forget” many
steps of our program’s execution until the condition is handled: the
call stack is unwound (or “untangled”, “shortened”). handler-bind
does not rewind the
stack. Let’s illustrate this.
For the sake of our demonstration, we will use the library
trivial-backtrace
, which you can install with Quicklisp:
(ql:quickload "trivial-backtrace")
It is a wrapper around the implementations’ primitives such as sb-debug:print-backtrace
.
Consider the following code: our main
function calls a chain of
functions which ultimately fail by signaling an error
. We handle the
error in the main function with hander-case
and print the backtrace.
(defun f0 ()
(error "oh no"))
(defun f1 ()
(f0))
(defun f2 ()
(f1))
(defun main ()
(handler-case (f2)
(error (c)
(format t "in main, we handle: ~a" c)
(trivial-backtrace:print-backtrace c))))
This is the backtrace (only the first frames):
CL-REPL> (main)
in main, we handle: oh no
Date/time: 2025-07-04-11:25!
An unhandled error condition has been signalled: oh no
Backtrace for: #<SB-THREAD:THREAD "repl-thread" RUNNING {1008695453}>
0: [...]
1: (TRIVIAL-BACKTRACE:PRINT-BACKTRACE ... )
2: (MAIN)
[...]
So far so good. It is trivial-backtrace
that prints the “Date/time” and the message “An unhandled error condition...”.
Now compare the stacktrace when we use handler-bind
:
(defun main-no-stack-unwinding ()
(handler-bind
((error (lambda (c)
(format t "in main, we handle: ~a" c)
(trivial-backtrace:print-backtrace c)
(return-from main-no-stack-unwinding))))
(f2)))
CL-REPL> (main-no-stack-unwinding)
in main, we handle: oh no
Date/time: 2025-07-04-11:32!
An unhandled error condition has been signalled: oh no
Backtrace for: #<SB-THREAD:THREAD "repl-thread" RUNNING {1008695453}>
0: ...
1: (TRIVIAL-BACKTRACE:PRINT-BACKTRACE ...)
2: ...
3: ...
4: (ERROR "oh no")
5: (F0)
6: (F1)
7: (MAIN-NO-STACK-UNWINDING)
That’s right: you can see all the call stack: from the main function
to the error through f1
and f0
. These two intermediate functions
were not present in the backtrace when we used handler-case
because,
as the error was signaled and bubbled up in the call stack, the stack
was unwound, and we lost information.
When to use which?
handler-case
is enough when you expect a situation to fail. For
example, in the context of an HTTP request, it is a common to anticipate a 400-ish error:
;; using the dexador library.
(handler-case (dex:get "http://bad-url.lisp")
(dex:http-request-failed (e)
;; For 4xx or 5xx HTTP errors: it's OK, this can happen.
(format *error-output* "The server returned ~D" (dex:response-status e))))
In other exceptional situations, we’ll surely want handler-bind
. For
example, when we want to handle what went wrong and we want to print a
backtrace, or if we want to invoke the debugger manually (see below)
and see exactly what happened.
Invoking the debugger manually
Suppose you handle a condition with handler-bind
, and your condition
object is bound to the c
variable (as in our examples
above). Suppose a parameter of yours, say *devel-mode*
, tells you
are not in production. It may be handy to fire the debugger on the
given condition. Use:
(invoke-debugger c)
In production, you can print the backtrace instead and have an error reporting tool like Sentry notify you.
Closing words
This is yet another CL feature I wish I had known earlier and learned by chance. I hope you learned a thing or two!
Joe Marshall — LLM failures
@2025-07-07 15:56 · 4 days agoI recently tried to get an LLM to solve two problems that I thought were well within its capabilities. I was suprised to find them unable to solve them.
Problem 1: Given a library of functions, re-order the function definitions in alphabetical order.
It can be useful to have the functions in alphabetical order so you can find them easily (assuming you are not constrained by any other ordering). The LLM was given a file of function definitions and was asked to put them in alphabetical order. It refused to do so, claiming that it was unable to determine the function boundaries because it had no model of the language syntax.
Fair enough, but a model of the language syntax isn't strictly necessary. Function definitions in most programming languages are easily identified: they are separated by blank lines, they start at the left margin, the body of the function is indented, the delimiters are balanced, etc. This is source code that was written by the LLM, so it is able to generate syntactically correct functions. It surprised me that it gave up so easily on the much simpler task of re-arranging the blocks of generated text.
Problem 2: Given the extended BNF grammar of the Go programming language, a) generate a series of small Go programs that illustrate the various grammar productions, b) generate a parser for the grammar.
This is a problem that I would have thought would be right up the LLM's alley. Although it easily generated twenty Go programs of varying complexity, it utterly failed to generate a parser that could handle them.
Converting a grammar to a parser is a common task, and I cannot believe that a parser for the Go language does not have several on-line examples. Furthemore taking a grammar as an input and producing a parser is a well-solved problem. The LLM made a quick attempt at generating a simple recursive descent parser (which is probably the easiest way to turn a grammar into a parser short of using a special tool), but the resultant parser could only handle the most trivial programs. When I asked the LLM to extend the parser to handle the more complex examples it had generated, it started hallucinating and getting lost in the weeds. I spent several hours redirecting the LLM and refining my prompts to help it along, but it never was able to make much progress beyond the simple parsing.
(I tried both Cursor with Claude Sonnet 4 and Gemini CLI with Gemini-2.5)
Both these problems uncovered surprising limitations to using LLMs to generate code. Both problems seem to me to be in the realm of problems that one would task to an LLM.
Joe Marshall — You Are The Compiler
@2025-06-30 18:15 · 11 days agoConsider a complex nested function call like
(foo (bar (baz x)) (quux y))
This is a tree of function calls. The outer call to foo
has two arguments, the result of the inner call to bar
and the result of the inner call to quux
. The inner
calls may themselves have nested calls.
One job of the compiler is to linearize this call tree into a sequential series of calls. So the compiler would generate some temporaries to hold the results of the inner calls, make each inner call in turn, and then make the outer call.
temp1 = baz(x) temp2 = bar(temp1) temp3 = quux(y) return foo (temp2, temp3)
Another job of the compiler is to arrange for each call to follow the calling conventions that define where the arguments are placed and where the results are returned. There may be additional tasks done at function call boundaries, for example, the system might insert interrupt checks after each call. These checks are abstracted away at the source code level. The compiler takes care of them automatically.
Sometimes, however, you want to want modify the calling conventions. For example, you might want to write in continuation passing style. Each CPS function will take an additional argument which is the continuation. The compiler won't know about this convention, so it will be incumbent on the programmer to write the code in a particular way.
If possible, a macro can help with this. The macro will ensure that the modified calling convention is followed. This will be less error prone than expecting the programmer to remember to write the code in a particular way.
The Go language has two glaring omissions in the standard calling conventions: no dynamic (thread local) variables and no error handling. Users are expected to impose their own calling conventions of passing an additional context argument between functions and returning error objects upon failures. The programmer is expected to write code at the call site to check the error object and handle the failure.
This is such a common pattern of usage that we can consider it to be the de facto calling convention of the language. Unfortunately, the compiler is unaware of this convention. It is up to the programmer to explicitly write code to assign the possible error object and check its value.
This calling convention breaks nested function calls. The user has to explicitly linearize the calls.
temp1, err1 := baz(ctx, x) if err1 != nil { return nil, err1 } temp2, err2 := bar(ctx, temp1) if err2 != nil { return nil, err2 } temp3, err3 := quux(ctx, y) if err2 != nil { return nil, err2 } result, err4 := foo(ctx, temp2, temp3) if err4 != nil { return nil, err4 } return result, nil
Golang completely drops the ball here. The convention of returning an error object and checking it is ubiquitous in the language, but there is no support for it in the compiler. The user ends up doing what is normally considered the compiler's job of linearizing nested calls and checking for errors. Of course users are less disciplined than the compiler, so unconventional call sequences and forgetting to handle errors are common.
Neil Munro — Ningle Tutorial 8: Mounting Middleware
@2025-06-29 11:30 · 12 days agoContents
- Part 1 (Hello World)
- Part 2 (Basic Templates)
- Part 3 (Introduction to middleware and Static File management)
- Part 4 (Forms)
- Part 5 (Environmental Variables)
- Part 6 (Database Connections)
- Part 7 (Envy Configuation Switching)
- Part 8 (Mouning Middleware)
Introduction
Welcome back to this Ningle tutorial series, in this part we are gonna have another look at some middleware, now that we have settings and configuration done there's another piece of middleware we might want to look at; application mounting
, many web frameworks have the means to use apps within other apps, you might want to do this because you have some functionality you use over and over again in many projects, it makes sense to make it into an app and simply include it in other apps. You might also might want to make applications available for others to use in their applications.
Which is exactly what we are gonna do here, we spent some time building a registration view, but for users we might want to have a full registration system that will have:
- Register
- Login
- Logout
- Account Verification
- Account Reset
- Account Deletion
Creating the auth app
We will begin by building the basic views that return a simple template and mount them into our main application, we will then fill the actual logic out in another tutorial. So, we will create a new Ningle project that has 6 views that simply handle get
requests, the important thing to bear in mind is that we will have to adjust the layout of our templates, we need our auth app to use its own templates, or use the templates of a parent app, this means we will have to namespace our templates, if you have use django before this will seem familiar.
Using my project builder set up a new project for our authentication application.
(nmunro:make-project #p"~/quicklisp/local-projects/ningle-auth/")
This will create a project skeleton, complete with an asd
file, a src
, and tests
directory. In the asd
file we need to add some packages (we will add more in a later tutorial).
:depends-on (:cl-dotenv
:clack
:djula
:envy-ningle
:mito
:ningle)
In the src/main.lisp
file, we will add the following:
1
2
3
4
5
6
7
8
9
10
11
12
13
14
15
16
17
18
19
20
21
22
23
24
25
26
27
28
29
30
31
32
33
34
35
36
37
38
39
40
41
42
43
44
45
46
47
48
49
50
51
52
53
(defpackage ningle-auth
(:use :cl)
(:export #:*app*
#:start
#:stop))
(in-package ningle-auth)
(defvar *app* (make-instance 'ningle:app))
(djula:add-template-directory (asdf:system-relative-pathname :ningle-auth "src/templates/"))
(setf (ningle:route *app* "/register")
(lambda (params)
(format t "Test: ~A~%" (mito:retrieve-by-sql "SELECT 2 + 3 AS result"))
(djula:render-template* "auth/register.html" nil :title "Register")))
(setf (ningle:route *app* "/login")
(lambda (params)
(djula:render-template* "auth/login.html" nil :title "Login")))
(setf (ningle:route *app* "/logout")
(lambda (params)
(djula:render-template* "auth/logout.html" nil :title "Logout")))
(setf (ningle:route *app* "/reset")
(lambda (params)
(djula:render-template* "auth/reset.html" nil :title "Reset")))
(setf (ningle:route *app* "/verify")
(lambda (params)
(djula:render-template* "auth/verify.html" nil :title "Verify")))
(setf (ningle:route *app* "/delete")
(lambda (params)
(djula:render-template* "auth/delete.html" nil :title "Delete")))
(defmethod ningle:not-found ((app ningle:<app>))
(declare (ignore app))
(setf (lack.response:response-status ningle:*response*) 404)
(djula:render-template* "error.html" nil :title "Error" :error "Not Found"))
(defun start (&key (server :woo) (address "127.0.0.1") (port 8000))
(djula:add-template-directory (asdf:system-relative-pathname :ningle-auth "src/templates/"))
(djula:set-static-url "/public/")
(clack:clackup
(lack.builder:builder (envy-ningle:build-middleware :ningle-auth/config *app*))
:server server
:address address
:port port))
(defun stop (instance)
(clack:stop instance))
Just as we did with our main application, we will need to create a src/config.lisp
:
1
2
3
4
5
6
7
8
9
10
11
12
13
14
15
(defpackage ningle-auth/config
(:use :cl :envy))
(in-package ningle-auth/config)
(dotenv:load-env (asdf:system-relative-pathname :ningle-auth ".env"))
(setf (config-env-var) "APP_ENV")
(defconfig :common
`(:application-root ,(asdf:component-pathname (asdf:find-system :ningle-auth))))
(defconfig |test|
`(:debug T
:middleware ((:session)
(:mito (:sqlite3 :database-name ,(uiop:getenv "SQLITE_DB_NAME"))))))
Now, I mentioned that the template files need to be organised in a certain way, we will start with the new template layout in our auth application, the directory structure should look like this:
➜ ningle-auth git:(main) tree .
.
├── ningle-auth.asd
├── README.md
├── src
│ ├── config.lisp
│ ├── main.lisp
│ └── templates
│ ├── ningle-auth
│ │ ├── delete.html
│ │ ├── login.html
│ │ ├── logout.html
│ │ ├── register.html
│ │ ├── reset.html
│ │ └── verify.html
│ ├── base.html
│ └── error.html
└── tests
└── main.lisp
So in your src/templates
directory there will be a directory called ningle-auth
and two files base.html
and error.html
, it is important that this structure is followed, as when the app is used as part of a larger app, we want to be able to layer templates, and this is how we do it.
base.html
1
2
3
4
5
6
7
8
9
10
11
12
13
14
<!doctype html>
<html lang="en">
<head>
<title>{{ title }}</title>
<link href="https://cdn.jsdelivr.net/npm/bootstrap@5.3.0/dist/css/bootstrap.min.css" rel="stylesheet">
</head>
<body>
<div class="container mt-4">
{% block content %}
{% endblock %}
</div>
<script src="https://cdn.jsdelivr.net/npm/bootstrap@5.3.0/dist/js/bootstrap.bundle.min.js"></script>
</body>
</html>
error.html
1
2
3
4
5
6
7
8
9
10
11
{% extends "base.html" %}
{% block content %}
<div class="container">
<div class="row">
<div class="col-12">
<h1>{{ error }}</h1>
</div>
</div>
</div>
{% endblock %}
Now the rest of the html files are similar, with only the title changing. Using the following html, create files for:
delete.html
1
2
3
4
5
6
7
8
9
10
11
{% extends "base.html" %}
{% block content %}
<div class="container">
<div class="row">
<div class="col-12">
<h1>Delete</h1>
</div>
</div>
</div>
{% endblock %}
login.html
1
2
3
4
5
6
7
8
9
10
11
{% extends "base.html" %}
{% block content %}
<div class="container">
<div class="row">
<div class="col-12">
<h1>Login</h1>
</div>
</div>
</div>
{% endblock %}
logout.html
1
2
3
4
5
6
7
8
9
10
11
{% extends "base.html" %}
{% block content %}
<div class="container">
<div class="row">
<div class="col-12">
<h1>Logout</h1>
</div>
</div>
</div>
{% endblock %}
register.html
1
2
3
4
5
6
7
8
9
10
11
{% extends "base.html" %}
{% block content %}
<div class="container">
<div class="row">
<div class="col-12">
<h1>Register</h1>
</div>
</div>
</div>
{% endblock %}
reset.html
1
2
3
4
5
6
7
8
9
10
11
{% extends "base.html" %}
{% block content %}
<div class="container">
<div class="row">
<div class="col-12">
<h1>Reset</h1>
</div>
</div>
</div>
{% endblock %}
verify.html
1
2
3
4
5
6
7
8
9
10
11
{% extends "base.html" %}
{% block content %}
<div class="container">
<div class="row">
<div class="col-12">
<h1>Verify</h1>
</div>
</div>
</div>
{% endblock %}
There is one final file to create, the .env
file! Even though this application wont typically run on its own, we will use one to test it is all working, since we did write src/config.lisp
afterall!
1
2
APP_ENV=test
SQLITE_DB_NAME=ningle-auth.db
Testing the auth app
Now that the auth application has been created we will test that it at least runs on its own, once we have confirmed this, we can integrate it into our main app. Like with our main application, we will load the system and run the start function that we defined.
(ql:quickload :ningle-auth)
To load "ningle-auth":
Load 1 ASDF system:
ningle-auth
; Loading "ningle-auth"
..................................................
[package ningle-auth/config].
(:NINGLE-AUTH)
(ningle-auth:start)
NOTICE: Running in debug mode. Debugger will be invoked on errors.
Specify ':debug nil' to turn it off on remote environments.
Woo server is started.
Listening on 127.0.0.1:8000.
#S(CLACK.HANDLER::HANDLER
:SERVER :WOO
:SWANK-PORT NIL
:ACCEPTOR #<BT2:THREAD "clack-handler-woo" {1203E4E3E3}>)
*
If this works correctly, you should be able to access the defined routes in your web browser, if not, and there is an error, check that another web server isn't running on port 8000 first! When you are able to access the simple routes from your web browser, we are ready to integrate this into our main application!
Integrating the auth app
Made it this far? Congratulations, we are almost at the end, I'm sure you'll be glad to know, there isn't all that much more to do, but we do have to ensure we follow the structure we set up in the auth app, which we will get to in just a moment, first, lets remember to add the ningle-auth
app to our dependencies in our project asd
file.
:depends-on (:cl-dotenv
:clack
:djula
:cl-forms
:cl-forms.djula
:cl-forms.ningle
:envy
:envy-ningle
:ingle
:mito
:mito-auth
:ningle
:ningle-auth) ;; add this
Next, we need to move most of our template files into a directory called main
, to make things easy, the only two templates we will not move are base.html
and error.html
; create a new directory src/templates/main
and put everything else in there.
For reference this is what your directory structure should look like:
➜ ningle-tutorial-project git:(main) tree .
.
├── ningle-tutorial-project.asd
├── ntp.db
├── README.md
├── src
│ ├── config.lisp
│ ├── forms.lisp
│ ├── main.lisp
│ ├── migrations.lisp
│ ├── models.lisp
│ ├── static
│ │ ├── css
│ │ │ └── main.css
│ │ └── images
│ │ ├── logo.jpg
│ │ └── lua.jpg
│ └── templates
│ ├── base.html
│ ├── error.html
│ └── main
│ ├── index.html
│ ├── login.html
│ ├── logout.html
│ ├── people.html
│ ├── person.html
│ └── register.html
└── tests
└── main.lisp
With the templates having been moved, we must find all areas in src/main.lisp
where we reference one of these templates and point to the new location, thankfully there's only 4 lines that need to be changed, the render-template* calls, below is what they should be changed to.
(djula:render-template* "main/index.html" nil :title "Home" :user user :posts posts)
(djula:render-template* "main/people.html" nil :title "People" :users users)
(djula:render-template* "main/person.html" nil :title "Person" :user user)
(djula:render-template* "main/register.html" nil :title "Register" :form form)
Here is a complete listing of the file in question.
(defpackage ningle-tutorial-project
(:use :cl :sxql)
(:import-from
:ningle-tutorial-project/forms
#:email
#:username
#:password
#:password-verify
#:register)
(:export #:start
#:stop))
(in-package ningle-tutorial-project)
(defvar *app* (make-instance 'ningle:app))
(setf (ningle:route *app* "/")
(lambda (params)
(let ((user (list :username "NMunro"))
(posts (list (list :author (list :username "Bob") :content "Experimenting with Dylan" :created-at "2025-01-24 @ 13:34")
(list :author (list :username "Jane") :content "Wrote in my diary today" :created-at "2025-01-24 @ 13:23"))))
(djula:render-template* "main/index.html" nil :title "Home" :user user :posts posts))))
(setf (ningle:route *app* "/people")
(lambda (params)
(let ((users (mito:retrieve-dao 'ningle-tutorial-project/models:user)))
(djula:render-template* "main/people.html" nil :title "People" :users users))))
(setf (ningle:route *app* "/people/:person")
(lambda (params)
(let* ((person (ingle:get-param :person params))
(user (first (mito:select-dao
'ningle-tutorial-project/models:user
(where (:or (:= :username person)
(:= :email person)))))))
(djula:render-template* "main/person.html" nil :title "Person" :user user))))
(setf (ningle:route *app* "/register" :method '(:GET :POST))
(lambda (params)
(let ((form (cl-forms:find-form 'register)))
(if (string= "GET" (lack.request:request-method ningle:*request*))
(djula:render-template* "main/register.html" nil :title "Register" :form form)
(handler-case
(progn
(cl-forms:handle-request form) ; Can throw an error if CSRF fails
(multiple-value-bind (valid errors)
(cl-forms:validate-form form)
(when errors
(format t "Errors: ~A~%" errors))
(when valid
(cl-forms:with-form-field-values (email username password password-verify) form
(when (mito:select-dao 'ningle-tutorial-project/models:user
(where (:or (:= :username username)
(:= :email email))))
(error "Either username or email is already registered"))
(when (string/= password password-verify)
(error "Passwords do not match"))
(mito:create-dao 'ningle-tutorial-project/models:user
:email email
:username username
:password password)
(ingle:redirect "/people")))))
(error (err)
(djula:render-template* "error.html" nil :title "Error" :error err))
(simple-error (csrf-error)
(setf (lack.response:response-status ningle:*response*) 403)
(djula:render-template* "error.html" nil :title "Error" :error csrf-error)))))))
(defmethod ningle:not-found ((app ningle:<app>))
(declare (ignore app))
(setf (lack.response:response-status ningle:*response*) 404)
(djula:render-template* "error.html" nil :title "Error" :error "Not Found"))
(defun start (&key (server :woo) (address "127.0.0.1") (port 8000))
(djula:add-template-directory (asdf:system-relative-pathname :ningle-tutorial-project "src/templates/"))
(djula:set-static-url "/public/")
(clack:clackup
(lack.builder:builder (envy-ningle:build-middleware :ningle-tutorial-project/config *app*))
:server server
:address address
:port port))
(defun stop (instance)
(clack:stop instance))
The final step we must complete is actually mounting our ningle-auth
application into our main app, which is thankfully quite easy. Mounting middleware exists for ningle
and so we can configure this in src/config.lisp
, to demonstrate this we will add it to our sqlite
config:
1
2
3
4
5
6
(defconfig |sqlite|
`(:debug T
:middleware ((:session)
(:mito (:sqlite3 :database-name ,(uiop:getenv "SQLITE_DB_NAME")))
(:mount "/auth" ,ningle-auth:*app*) ;; This line!
(:static :root ,(asdf:system-relative-pathname :ningle-tutorial-project "src/static/") :path "/public/"))))
You can see on line #5 that a new mount
point is being defined, we are mounting all the routes that ningle-auth
has, onto the /auth
prefix. This means that, for example, the /register
route in ningle-auth
will actually be accessed /auth/register
.
If you can check that you can access all the urls to confirm this works, then we have assurances that we are set up correctly, however we need to come back to the templates one last time.
The reason we changed the directory structure, because ningle-auth is now running in the context of our main app, we can actually override the templates, so if we wanted to, in our src/templates
directory, we could create a ningle-auth
directory and create our own register.html
, login.html
, etc, allowing us to style and develop our pages as we see fit, allowing complete control to override, if that is our wish. By NOT moving the base.html
and error.html
files, we ensure that templates from another app can inherit our styles and layouts in a simple and predictable manner.
Conclusion
Wow, what a ride... Thanks for sticking with it this month, although, next month isn't going to be much easier as we begin to develop a real authentication application for use in our microblog app! As always, I hope you have found this helpful and you have learned something.
In this tutorial you should be able to:
- Explain what mounting an application means
- Describe how routes play a part in mounting an application
- Justify why you might mount an application into another
- Develop and mount an application inside another
Github
- The link for this tutorials code is available here.
- The link for the auth app code is available here.
Resources
Joe Marshall — No Error Handling For You
@2025-06-11 14:25 · 30 days agoAccording to the official Go blog, there are no plans to fix the (lack of) error handling in Go. Typical. Of course they recognize the problem, and many people have suggested solutions, but no one solution seems to be obviously better than the others, so they are going to do nothing. But although no one solution appears obviously better than the others, it's pretty clear that the status quo is worse than any of the proposed solutions.
But the fundamental problem isn't error handling. The fundamental problem is that the language cannot be extended and modified by the user. Error handling requires a syntactic change to the language, and changes to the language have to go through official channels.
If Go had a macro system, people could write their own error handling system. Different groups could put forward their proposals independently as libraries, and you could choose the error handling library that best suited your needs. No doubt a popular one would eventually become the de facto standard.
But Go doesn't have macros, either. So you are stuck with
limitations that are baked into the language. Naturally, there will
be plenty of people who will argue that this is a good thing. At
least the LLMs will have a lot of training data for if err !=
nil
.
Joe Marshall — Continuation-passing-style in Golang
@2025-06-10 07:00 · 31 days agoI had a requirement change come in the other day. It was for a golang program that made some REST calls to an API. The change was that if a user did not supply credentials, the program should still be able to call those parts of the REST API that could handle anonymous requests. If the user supplied credentials, then all calls should use them. If the user did not supply credentials, but attempted to call a part of the API that required them, the program would panic with missing credentials and exit.
In Lisp, this is good use case for continuation-passing-style. The routine that fetches the credentials could take two continuations, one to call with the user-supplied credentials and one to call if no credentials are found. The second continuation could take a condition object that indicates why the credentials were not found.
A caller requesting credentials could call this routine with two continuations. The first would be a lexical closure that accepted the credentials as an argument and invoked the relevant API call. The second could either be a first-class function that accepted the condition and signalled it (and so the credentials would be required) or it could be a lexical closure that just invoked the API without credentials (and so make an anonymous request).
This separates the concerns nicely. The code that fetches the credentials doesn't have to know whether the credentials are ultimately required or optional. The code that uses the credentials doesn't have to know how they were fetched or where they are fetched from.
But I was working in Golang, not Lisp. Nevertheless, you can write a limited form of continuation-passing-style in Golang. Golang supports first-class functions and lexical closures, so you can pass these down as a continuations. But there are a couple of problems.
First, there is no tail recursion in Golang. This means that a stack frame is pushed on each call regardless of whether it is a continuation or not. You will run out of stack space if you call several continuation-passing-style routines in a row, especially if they end up looping. Each subroutine call pushes an "identity" stack frame that just returns what is returned to it, and these pile up. When you write continuation-passing-style code in Golang, you have to be careful to return to direct style often enough to pop these accumulating identity frames off the stack.
Second, Golang distinguishes between expressions that return a value and statements that do not. The function definition syntax is used for both, the difference being whether you declare a return type or not. A continuation-passing-style function returns a value of the same type as the continuation returns. For this we use a generic function that takes a type parameter for the return type:
func foo[T any](cont func(int) T) T { ... do something ... return cont(...some integer value...) }
But what if the continuation is a statement that does not return a value? In this case, we don't want a return type at all.
func fooVoid (cont func(int)) { ... do something ... cont(...some integer value...) return }
The problem here is that we now need two versions of every continuation-passing-style routine, one that returns a value and one that does not, and we have to manually choose which version to use at each call site. This is tedious and error-prone. It would be nice to have a "void" type that acts as a placeholder to indicate that no actual return value is expected.
Joe Marshall — Generating Images
@2025-06-09 17:04 · 32 days agoIt was suprisingly hard to persuade the image generator to generate a female Common Lisp alien. Asking for a female Lisp alien just produced some truly disturbing images. Evertually I uploaded the original alien and told it to add a pink bow and eyelashes, but it kept adding an extra arm to hold the flag. I had to switch to a different LLM model that eventually got it.
Joe Marshall — Avoiding Management
@2025-06-09 16:00 · 32 days agoYou can probably guess from prior blog posts that I am not a manager. I have made it clear in my last several gigs that I have no interest in management and that I only wish to be an individual contributor. I've managed before and I don't like it. The perqs - more control over the project, more money, a bigger office - don't even come close to the disadvantages - more meetings, less contact with the code, dealing with people problems, and so on.
The worst part of managing is dealing with people. In any group of people, there is some median level of performance, and by definition of the median, half the people are below that level. And one is at the bottom. He's the one who is always late, always had the bad luck, never checks in code, and so on. He struggles. Perhaps the right thing to do is to let him go, but I've been there. I've had jobs where the fit wasn't right and I wasn't performing at my best. I empathize. The last thing you need when you're in that position is the sword of Damocles hanging over your head. It's a horrible position to be in and I don't want to exacerbate it. I'll gladly forego the perqs of management to avoid being in the situation where I have to fire someone or even threaten to fire them.
But I do like to help people. I like to mentor and coach. I can usually keep the big picture in my head and I can help people find the places where they can be most effective. I'm pretty comfortable being a senior engineer, a technical lead, or an architect.
Scheduling is hard. I've got a rule of thumb for estimating how long a project will take. I take the best estimate I can get from the team, and then I triple it. If I think it will take two weeks, I tell upper management it will take six. It is almost always a realistic estimate - I'm not padding or sandbagging. When I get the time I ask for, I almost always deliver on time. But manegement rarely likes to hear the word "six" when they were hoping for "two".
There is always overhead that is hard to account for. You come in some day and find that your infrastructure is down and you have to spend a whole day fixing it. Or you need to coordinate with another team and it takes two days to get everyone on the same page. Or you discover that the API you were depending on hasn't really been implemented yet, and you have to spend a day or two writing a workaroud. These add up. As a contributor, I'm 100% busy developing and putting out fires, but managers only want to count the time spent developing.
The best managers I had didn't manage. They didn't assign work, or try to tell me what to do or try to direct the project. Instead, they acted as my off-board memory. I could rathole on some problem and when I solved it and came up for air, they would be there to remind me what I was in the middle of to begin with. They would keep track of the ultimate goals of the project and how we were progressing towards them, but they would not try to direct the activity. They trusted that I knew how to do my job. They acted as facilitators and enablers, not as managers. They would interface with upper management. They would handle the politics. They would justify the six weeks I asked for.
So I prefer to be an individual contributor and I avoid management like the plague. Programming makes me happy, and I don't need the stress of management.
Neil Munro — Ningle Tutorial 7: Envy Configuration Switching
@2025-05-31 21:30 · 40 days agoContents
- Part 1 (Hello World)
- Part 2 (Basic Templates)
- Part 3 (Introduction to middleware and Static File management)
- Part 4 (Forms)
- Part 5 (Environmental Variables)
- Part 6 (Database Connections)
- Part 7 (Envy Configuation Switching)
- Part 8 (Mouning Middleware)
Introduction
Welcome back, in this tutorial we will look at how to simplify the complexities introduced last time. We had three different versions of our application, depending on which SQL database we wanted to use, this is hardly an ideal situation, we might want to run SQLite on one environment and PostgreSQL on another, it does not make sense to have to edit code to change such things, we should have code that is generalised and some configuration (like environmental variables) can provide the system with the connection information.
We want to separate our application configuration from our application logic, in software development we might build an application and have different environments in which is can be deployed, and different cloud providers/environments might have different capabilities, for example some providers provide PostgreSQL and others MySQL. As application designers we do not want to concern ourselves with having to patch our application based whatever an environment has provided, it would be better if we had a means by which we could read in how we connect to our databases and defer to that.
This type of separation is very common, in fact it is this separation that ningle
itself if for! Just as now we are creating a means to connect to a number of different databases based on config, ningle
allows us to run on a number of different webservers, without ningle
we would have to write code directly in the way a web server expects, ningle
allows us to write more generalised code.
Enter envy, a package that allows us to define different application configurations. Envy will allow us to set up different configurations and switch them based on an environmental variable, just like we wanted. Using this allows us to remove all of our database specific connection code and read it from a configuration, the configuration of which can be changed, the application can be restarted and everything should just work.
We have a slight complication in that we have our migration code, so we will need a way to also extract the active settings, but I wrote a package to assist in this envy-ningle, we will use both these packages to clean up our code.
Installing Packages
To begin with we will need to ensure we have installed and added the packages we need to our project asd file, there are two that we will be installing:
Note: My package (envy-ningle) is NOT in quicklisp, so you will need to clone it using git
into your local-packages
directory.
Once you have acquired the packages, as normal you will need to add them in the :depends-on
section.
:depends-on (:clack
:cl-dotenv
:djula
:cl-forms
:cl-forms.djula
:cl-forms.ningle
:envy ;; Add this
:envy-ningle ;; Add this
:ingle
:mito
:mito-auth
:ningle)
Writing Application Configs
config.lisp
We must write our application configs somewhere, so we will do that in src/config.lisp
, as always when adding a new file to our application we must ensure it is added to the asd
file, in the :components section
. This will ensure the file will be loaded and compiled when the system is loaded.
:components ((:module "src"
:components
((:file "config") ;; Add this
(:file "models")
(:file "migrations")
(:file "forms")
(:file "main"))))
So we should write this file now!
As normal we set up a package, declare what packages we will use (:use :cl :envy)
and set the active package to this one. There's some conventions we must follow using this that may seem unimportant at first, but actually are, specifically the |sqlite|
, |mysql|
, and |postgresql|
they must include the |
surrounding the name, (although the name doesn't have to be sqlite
, mysql
, or postgresql
, those are just what I used based on the last tutorial).
(defpackage ningle-tutorial-project/config
(:use :cl :envy))
(in-package ningle-tutorial-project/config)
We will start by loading the .env
file using the dotenv
package, we will remove it from our main.lisp
file a little later, but we need to include it here, next we will inform envy of what the name of the environmental variable is that will be used to switch config, in this case APP_ENV
.
(dotenv:load-env (asdf:system-relative-pathname :ningle-tutorial-project ".env"))
(setf (config-env-var) "APP_ENV")
This means that in your .env
file you should add the following:
Note: I am using the sqlite config here, but you can use any of the configs below.
APP_ENV=sqlite
We can define a "common" set of configs using the :common
label, this differs from the other labels that use the |
to surround them. The :common
config isn't one that will actually be used, it just provides a place to store the, well, common, configuration. While we don't yet necessarily have any shared config at this point, it is important to understand how to achieve it. In this example we set an application-root
that all configs will share.
In envy
we use the defconfig
macro
to define a config. Configs take a name, and a list
of items. There is a shared configuration which is called :common
, that any number of other custom configs that inherit from, their names are arbitary, but must be surrounded by |
, for example |staging|
, or |production|
.
This is the :common
we will use in this tutorial:
(defconfig :common
`(:application-root ,(asdf:component-pathname (asdf:find-system :ningle-tutorial-project))))
We can now define our actual configs, our "development" config will be sqlite
, which will define our database connection, however, because mito
defines database connections as middleware, we can define the middleware section in our config. Each config will have a different middleware section. Unfortunately there will be some repetition with the (:session)
and (:static ...)
middleware sections.
(defconfig |sqlite|
`(:debug T
:middleware ((:session)
(:mito (:sqlite3 :database-name ,(uiop:getenv "SQLITE_DB_NAME")))
(:static :root ,(asdf:system-relative-pathname :ningle-tutorial-project "src/static/") :path "/public/"))))
For our MySQL
config we have this:
(defconfig |mysql|
`(:middleware ((:session)
(:mito (:mysql
:database-name ,(uiop:native-namestring (uiop:parse-unix-namestring (uiop:getenv "MYSQL_DB_NAME")))
:username ,(uiop:getenv "MYSQL_USER")
:password ,(uiop:getenv "MYSQL_PASSWORD")
:host ,(uiop:getenv "MYSQL_ADDRESS")
:port ,(parse-integer (uiop:getenv "MYSQL_PORT"))))
(:static :root ,(asdf:system-relative-pathname :ningle-tutorial-project "src/static/") :path "/public/"))))
And finally our PostgreSQL
:
(defconfig |postgresql|
`(:middleware ((:session)
(:mito (:postgres
:database-name ,(uiop:native-namestring (uiop:parse-unix-namestring (uiop:getenv "POSTGRES_DB_NAME")))
:username ,(uiop:getenv "POSTGRES_USER")
:password ,(uiop:getenv "POSTGRES_PASSWORD")
:host ,(uiop:getenv "POSTGRES_ADDRESS")
:port ,(parse-integer (uiop:getenv "POSTGRES_PORT"))))
(:static :root ,(asdf:system-relative-pathname :ningle-tutorial-project "src/static/") :path "/public/"))))
None of this should be especially new, this middleware section should be familiar from last time, simply wrapped up in the envy:defconfig
macro
.
Here is the file in its entirety:
(defpackage ningle-tutorial-project/config
(:use :cl :envy))
(in-package ningle-tutorial-project/config)
(dotenv:load-env (asdf:system-relative-pathname :ningle-tutorial-project ".env"))
(setf (config-env-var) "APP_ENV")
(defconfig :common
`(:application-root ,(asdf:component-pathname (asdf:find-system :ningle-tutorial-project))))
(defconfig |sqlite|
`(:debug T
:middleware ((:session)
(:mito (:sqlite3 :database-name ,(uiop:getenv "SQLITE_DB_NAME")))
(:static :root ,(asdf:system-relative-pathname :ningle-tutorial-project "src/static/") :path "/public/"))))
(defconfig |mysql|
`(:middleware ((:session)
(:mito (:mysql
:database-name ,(uiop:native-namestring (uiop:parse-unix-namestring (uiop:getenv "MYSQL_DB_NAME")))
:username ,(uiop:getenv "MYSQL_USER")
:password ,(uiop:getenv "MYSQL_PASSWORD")
:host ,(uiop:getenv "MYSQL_ADDRESS")
:port ,(parse-integer (uiop:getenv "MYSQL_PORT"))))
(:static :root ,(asdf:system-relative-pathname :ningle-tutorial-project "src/static/") :path "/public/"))))
(defconfig |postgresql|
`(:middleware ((:session)
(:mito (:postgres
:database-name ,(uiop:native-namestring (uiop:parse-unix-namestring (uiop:getenv "POSTGRES_DB_NAME")))
:username ,(uiop:getenv "POSTGRES_USER")
:password ,(uiop:getenv "POSTGRES_PASSWORD")
:host ,(uiop:getenv "POSTGRES_ADDRESS")
:port ,(parse-integer (uiop:getenv "POSTGRES_PORT"))))
(:static :root ,(asdf:system-relative-pathname :ningle-tutorial-project "src/static/") :path "/public/"))))
main.lisp
As mentioned, we need to do some cleanup in our main.lisp
, the first is to remove the dotenv
code that has been moved into the config.lisp
file, but we will also need to take advantage of the envy-ningle
package to load the active configuration into the lack
builder code.
To remove the dotenv
code:
(defvar *app* (make-instance 'ningle:app))
;; remove the line below
(dotenv:load-env (asdf:system-relative-pathname :ningle-tutorial-project ".env"))
(setf (ningle:route *app* "/")
Now to edit the start
function, it should look like the following:
(defun start (&key (server :woo) (address "127.0.0.1") (port 8000))
(djula:add-template-directory (asdf:system-relative-pathname :ningle-tutorial-project "src/templates/"))
(djula:set-static-url "/public/")
(clack:clackup
(lack.builder:builder (envy-ningle:build-middleware :ningle-tutorial-project/config *app*))
:server server
:address address
:port port))
As you can see, all of the previous middleware code that had to be changed if you wanted to switch databases, is now a single line, because envy
loads the config based on the environmental variable, the envy-ningle:build-middleware
function will then read that config and insert the middleware into the application. I hope you will agree that it is much simpler and makes your application much easier to manage.
If you are not yet convinced and you think you're fine to keep things as they were, consider that we have duplicated our database connection logic in migrations.lisp
and if we decide we do need to change our connection we have to do it in two places, possibly more if we have many models and want to break the code up.
migrations.lisp
We will use the same structure for how we loaded configuration in our main.lisp
file, the way we use envy-ningle
is different, previously we called the build-middleware
function, which is designed to place the config middleware into the lack
builder, here we want to get only the database connection information and thus we will use the extract-mito-config
(admittedly not the best name), to get the database connection information and use it in mito:connect-toplevel
.
(defun migrate ()
"Explicitly apply migrations when called."
(format t "Applying migrations...~%")
(multiple-value-bind (backend args) (envy-ningle:extract-mito-config :ningle-tutorial-project/config)
(unless backend
(error "No :mito middleware config found in ENVY config."))
(apply #'mito:connect-toplevel backend args)
(mito:ensure-table-exists 'ningle-tutorial-project/models:user)
(mito:migrate-table 'ningle-tutorial-project/models:user)
(mito:disconnect-toplevel)
(format t "Migrations complete.~%")))
As you can see here, we use multiple-value-bind
to get the "backend" (which will be one of the three supported SQL databases), and then the arguments that backend expects. If there isn't a backend, an error is thrown, if there is, we call apply
on the mito:connect-toplevel
with the "backend" and "args" values.
Testing The Config Switching
Now that all the code has been written, we will want to test it all works. The simplest way to do this is while the value of "APP_ENV" in your .env
file is "sqlite", run the migrations.
(ningle-tutorial-project/migrations:migrate)
You should see the sqlite specific output, if that works, we can then change the value of "APP_ENV" to be "mysql" or "postgresql", whichever you have available to you, and we can run the migrations again.
(ningle-tutorial-project/migrations:migrate)
This time we would expect to see different sql output, and if you do, you can confirm that the configurating switching is working as expected.
Conclusion
I hope you found that helpful, and that you agree that it's better to separate our configuration from our actual application code.
To recap, after working your way though this tutorial you should be able to:
- Explain what configuration switching is
- Explain why configuration is important
- Discuss the reasons for separating configuration from application code
- Implement your own configurations for applications you write
Github
- The link for this tutorials code is available here.
Resources
Joe Marshall — Vibe Coding, A Kind of Success
@2025-05-30 07:00 · 42 days agoYeah, these posts about vibe coding are a bit tedious, but I got an interesting result. My hypothesis about vibe coding in Common Lisp was that there is simply not enough training data. But the most popular lisp is not Common Lisp but Emacs Lisp. It should have enough training data. So I tried vibe coding an application in Emacs Lisp. Again I used minesweeper as the example.
The model was Claude 3.7 Sonnet and I used VSCode in "Agent" mode. It generated a "minesweeper.el" file with 455 lines of code. There were some errors when I tried to run it, so I simply copied the error message into the AI prompt and let the VSCode agent fix it. After a couple of iterations, the code seems to work. It isn't the most polished minsweeper clone, but it is sort of a serviceable game.
So I guess the conclusion is that vibe coding works in Emacs Lisp. The result isn't all that slick, but what do expect from an Emacs game? This tends to support the hypothesis that you need ample training data.
Here is the code:
;;; minesweeper.el --- A Minesweeper game for Emacs -*- lexical-binding: t -*- ;; Author: AI Assistant ;; Keywords: games ;; Version: 1.0 ;; Package-Requires: ((emacs "24.3")) ;;; Commentary: ;; This package implements the classic Minesweeper game for Emacs. ;; Use M-x minesweeper to start the game. ;;; Code: (require 'cl-lib) ;;; Constants and Variables (defvar minesweeper-mode-map (let ((map (make-sparse-keymap))) (define-key map (kbd "<down>") 'minesweeper-move-down) (define-key map (kbd "<up>") 'minesweeper-move-up) (define-key map (kbd "<left>") 'minesweeper-move-left) (define-key map (kbd "<right>") 'minesweeper-move-right) (define-key map (kbd "RET") 'minesweeper-reveal) (define-key map (kbd "SPC") 'minesweeper-flag) (define-key map (kbd "r") 'minesweeper-restart) (define-key map (kbd "q") 'minesweeper-quit) map) "Keymap for ‘minesweeper-mode'.") (defgroup minesweeper nil "Minesweeper game for Emacs." :group 'games) (defcustom minesweeper-board-width 10 "Width of the Minesweeper game board." :type 'integer :group 'minesweeper) (defcustom minesweeper-board-height 10 "Height of the Minesweeper game board." :type 'integer :group 'minesweeper) (defcustom minesweeper-mine-count 15 "Number of mines on the Minesweeper board." :type 'integer :group 'minesweeper) (defface minesweeper-hidden-face '((t :background "gray80" :box (:line-width -1 :color "gray50"))) "Face for hidden cells." :group 'minesweeper) (defface minesweeper-flag-face '((t :background "gray80" :foreground "red" :weight bold :box (:line-width -1 :color "gray50"))) "Face for flagged cells." :group 'minesweeper) (defface minesweeper-mine-face '((t :background "red" :foreground "black" :weight bold :box (:line-width -1 :color "gray50"))) "Face for mine cells." :group 'minesweeper) (defface minesweeper-empty-face '((t :background "gray95" :box (:line-width -1 :color "gray50"))) "Face for empty revealed cells." :group 'minesweeper) (defface minesweeper-number-1-face '((t :background "gray95" :foreground "blue" :weight bold :box (:line-width -1 :color "gray50"))) "Face for cells with 1 adjacent mine." :group 'minesweeper) (defface minesweeper-number-2-face '((t :background "gray95" :foreground "green" :weight bold :box (:line-width -1 :color "gray50"))) "Face for cells with 2 adjacent mines." :group 'minesweeper) (defface minesweeper-number-3-face '((t :background "gray95" :foreground "red" :weight bold :box (:line-width -1 :color "gray50"))) "Face for cells with 3 adjacent mines." :group 'minesweeper) (defface minesweeper-number-4-face '((t :background "gray95" :foreground "purple" :weight bold :box (:line-width -1 :color "gray50"))) "Face for cells with 4 adjacent mines." :group 'minesweeper) (defface minesweeper-number-5-face '((t :background "gray95" :foreground "maroon" :weight bold :box (:line-width -1 :color "gray50"))) "Face for cells with 5 adjacent mines." :group 'minesweeper) (defface minesweeper-number-6-face '((t :background "gray95" :foreground "turquoise" :weight bold :box (:line-width -1 :color "gray50"))) "Face for cells with 6 adjacent mines." :group 'minesweeper) (defface minesweeper-number-7-face '((t :background "gray95" :foreground "black" :weight bold :box (:line-width -1 :color "gray50"))) "Face for cells with 7 adjacent mines." :group 'minesweeper) (defface minesweeper-number-8-face '((t :background "gray95" :foreground "gray50" :weight bold :box (:line-width -1 :color "gray50"))) "Face for cells with 8 adjacent mines." :group 'minesweeper) (defvar minesweeper-buffer-name "*Minesweeper*" "Name of the Minesweeper game buffer.") (defvar minesweeper-board nil "The game board. Each cell is a list of the form (MINE-P REVEALED-P FLAGGED-P MINE-COUNT).") (defvar minesweeper-game-over nil "Whether the current game is over.") (defvar minesweeper-game-won nil "Whether the current game is won.") (defvar minesweeper-flags-placed 0 "Number of flags placed on the board.") (defvar minesweeper-current-pos '(0 . 0) "Current cursor position as (ROW . COL).") ;;; Game Functions (defun minesweeper-init-board () "Initialize the game board." (setq minesweeper-board (make-vector minesweeper-board-height nil)) (let ((board-cells (* minesweeper-board-width minesweeper-board-height)) (mine-positions (make-vector (* minesweeper-board-width minesweeper-board-height) nil))) ;; Initialize all cells (dotimes (row minesweeper-board-height) (let ((row-vec (make-vector minesweeper-board-width nil))) (dotimes (col minesweeper-board-width) (aset row-vec col (list nil nil nil 0))) ; (mine-p revealed-p flagged-p mine-count) (aset minesweeper-board row row-vec))) ;; Randomly place mines (dotimes (i minesweeper-mine-count) (let ((pos (random board-cells))) (while (aref mine-positions pos) (setq pos (random board-cells))) (aset mine-positions pos t) (let* ((row (/ pos minesweeper-board-width)) (col (% pos minesweeper-board-width)) (cell (aref (aref minesweeper-board row) col))) (setcar cell t)))) ; Set mine-p to t ;; Calculate adjacent mine counts (dotimes (row minesweeper-board-height) (dotimes (col minesweeper-board-width) (unless (car (aref (aref minesweeper-board row) col)) ; Skip if it's a mine (let ((count 0)) (dolist (r (list -1 0 1)) (dolist (c (list -1 0 1)) (unless (and (= r 0) (= c 0)) (let ((new-row (+ row r)) (new-col (+ col c))) (when (and (>= new-row 0) (< new-row minesweeper-board-height) (>= new-col 0) (< new-col minesweeper-board-width)) (when (car (aref (aref minesweeper-board new-row) new-col)) (setq count (1+ count)))))))) (setcar (nthcdr 3 (aref (aref minesweeper-board row) col)) count)))))) (setq minesweeper-game-over nil minesweeper-game-won nil minesweeper-flags-placed 0 minesweeper-current-pos '(0 . 0))) (defun minesweeper-get-cell (row col) "Get the cell at ROW and COL." (aref (aref minesweeper-board row) col)) (cl-defun minesweeper-reveal (row col) "Reveal the cell at ROW and COL." (interactive (if current-prefix-arg (list (read-number "Row: ") (read-number "Column: ")) (list (car minesweeper-current-pos) (cdr minesweeper-current-pos)))) (when minesweeper-game-over (message "Game over. Press 'r' to restart.") (cl-return-from minesweeper-reveal nil)) (let* ((cell (minesweeper-get-cell row col)) (mine-p (nth 0 cell)) (revealed-p (nth 1 cell)) (flagged-p (nth 2 cell)) (mine-count (nth 3 cell))) (when flagged-p (cl-return-from minesweeper-reveal nil)) (when revealed-p (cl-return-from minesweeper-reveal nil)) (setcar (nthcdr 1 cell) t) ; Set revealed-p to t (if mine-p (progn (setq minesweeper-game-over t) (minesweeper-reveal-all-mines) (minesweeper-draw-board) (message "BOOM! Game over.")) ;; Reveal adjacent cells if this is an empty cell (when (= mine-count 0) (dolist (r (list -1 0 1)) (dolist (c (list -1 0 1)) (unless (and (= r 0) (= c 0)) (let ((new-row (+ row r)) (new-col (+ col c))) (when (and (>= new-row 0) (< new-row minesweeper-board-height) (>= new-col 0) (< new-col minesweeper-board-width)) (minesweeper-reveal new-row new-col))))))) (minesweeper-check-win))) (minesweeper-draw-board)) (cl-defun minesweeper-flag (row col) "Toggle flag on cell at ROW and COL." (interactive (if current-prefix-arg (list (read-number "Row: ") (read-number "Column: ")) (list (car minesweeper-current-pos) (cdr minesweeper-current-pos)))) (when minesweeper-game-over (message "Game over. Press 'r' to restart.") (cl-return-from minesweeper-flag nil)) (let* ((cell (minesweeper-get-cell row col)) (revealed-p (nth 1 cell)) (flagged-p (nth 2 cell))) (when revealed-p (cl-return-from minesweeper-flag nil)) (if flagged-p (progn (setcar (nthcdr 2 cell) nil) ; Remove flag (setq minesweeper-flags-placed (1- minesweeper-flags-placed))) (setcar (nthcdr 2 cell) t) ; Add flag (setq minesweeper-flags-placed (1+ minesweeper-flags-placed)))) (minesweeper-draw-board)) (defun minesweeper-reveal-all-mines () "Reveal all mines on the board." (dotimes (row minesweeper-board-height) (dotimes (col minesweeper-board-width) (let* ((cell (minesweeper-get-cell row col)) (mine-p (nth 0 cell))) (when mine-p (setcar (nthcdr 1 cell) t)))))) ; Set revealed-p to t (defun minesweeper-check-win () "Check if the game is won." (let ((all-non-mines-revealed t)) (dotimes (row minesweeper-board-height) (dotimes (col minesweeper-board-width) (let* ((cell (minesweeper-get-cell row col)) (mine-p (nth 0 cell)) (revealed-p (nth 1 cell))) (when (and (not mine-p) (not revealed-p)) (setq all-non-mines-revealed nil))))) (when all-non-mines-revealed (setq minesweeper-game-over t minesweeper-game-won t) (message "You win!") (minesweeper-flag-all-mines)))) (defun minesweeper-flag-all-mines () "Flag all mines on the board." (dotimes (row minesweeper-board-height) (dotimes (col minesweeper-board-width) (let* ((cell (minesweeper-get-cell row col)) (mine-p (nth 0 cell)) (flagged-p (nth 2 cell))) (when (and mine-p (not flagged-p)) (setcar (nthcdr 2 cell) t)))))) ;;; UI Functions (defun minesweeper-draw-cell (row col) "Draw the cell at ROW and COL." (let* ((cell (minesweeper-get-cell row col)) (mine-p (nth 0 cell)) (revealed-p (nth 1 cell)) (flagged-p (nth 2 cell)) (mine-count (nth 3 cell)) (char " ") (face 'minesweeper-hidden-face) (current-p (and (= row (car minesweeper-current-pos)) (= col (cdr minesweeper-current-pos))))) (cond (flagged-p (setq char "F") (setq face 'minesweeper-flag-face)) (revealed-p (cond (mine-p (setq char "*") (setq face 'minesweeper-mine-face)) ((= mine-count 0) (setq char " ") (setq face 'minesweeper-empty-face)) (t (setq char (number-to-string mine-count)) (setq face (intern (format "minesweeper-number-%d-face" mine-count)))))) (t (setq char " ") (setq face 'minesweeper-hidden-face))) (insert (propertize char 'face face)) (when current-p (put-text-property (1- (point)) (point) 'cursor t)))) (defun minesweeper-draw-board () "Draw the game board." (let ((inhibit-read-only t) (old-point (point))) (erase-buffer) ;; Draw header (insert (format "Minesweeper: %d mines, %d flags placed\n\n" minesweeper-mine-count minesweeper-flags-placed)) ;; Draw column numbers (insert " ") (dotimes (col minesweeper-board-width) (insert (format "%d" (% col 10)))) (insert "\n") ;; Draw top border (insert " ") (dotimes (col minesweeper-board-width) (insert "-")) (insert "\n") ;; Draw board rows (dotimes (row minesweeper-board-height) (insert (format "%d|" (% row 10))) (dotimes (col minesweeper-board-width) (minesweeper-draw-cell row col)) (insert "|\n")) ;; Draw bottom border (insert " ") (dotimes (col minesweeper-board-width) (insert "-")) (insert "\n\n") ;; Draw status (cond (minesweeper-game-won (insert "You won! Press 'r' to restart or 'q' to quit.")) (minesweeper-game-over (insert "Game over! Press 'r' to restart or 'q' to quit.")) (t (insert "Press 'r' to restart, 'q' to quit.\n") (insert "Use arrows to move, ENTER to reveal, SPACE to toggle flag."))) ;; Restore point or set to position of cursor (goto-char (point-min)) (let ((result (text-property-search-forward 'cursor))) (if result (goto-char (prop-match-beginning result)) (goto-char old-point))))) ;;; Movement Functions (defun minesweeper-move-up () "Move cursor up." (interactive) (let ((row (car minesweeper-current-pos)) (col (cdr minesweeper-current-pos))) (when (> row 0) (setq minesweeper-current-pos (cons (1- row) col)) (minesweeper-draw-board)))) (defun minesweeper-move-down () "Move cursor down." (interactive) (let ((row (car minesweeper-current-pos)) (col (cdr minesweeper-current-pos))) (when (< row (1- minesweeper-board-height)) (setq minesweeper-current-pos (cons (1+ row) col)) (minesweeper-draw-board)))) (defun minesweeper-move-left () "Move cursor left." (interactive) (let ((row (car minesweeper-current-pos)) (col (cdr minesweeper-current-pos))) (when (> col 0) (setq minesweeper-current-pos (cons row (1- col))) (minesweeper-draw-board)))) (defun minesweeper-move-right () "Move cursor right." (interactive) (let ((row (car minesweeper-current-pos)) (col (cdr minesweeper-current-pos))) (when (< col (1- minesweeper-board-width)) (setq minesweeper-current-pos (cons row (1+ col))) (minesweeper-draw-board)))) ;;; Game Management Functions (defun minesweeper-restart () "Restart the Minesweeper game." (interactive) (minesweeper-init-board) (minesweeper-draw-board)) (defun minesweeper-quit () "Quit the Minesweeper game." (interactive) (kill-buffer minesweeper-buffer-name)) (define-derived-mode minesweeper-mode special-mode "Minesweeper" "Major mode for playing Minesweeper." (setq buffer-read-only t) (setq-local cursor-type nil) (buffer-disable-undo)) ;;;###autoload (defun minesweeper () "Start a new game of Minesweeper." (interactive) (switch-to-buffer minesweeper-buffer-name) (minesweeper-mode) (minesweeper-init-board) (minesweeper-draw-board)) (provide 'minesweeper) ;;; minesweeper.el ends here
To run it, you can save the code to a file named "minesweeper.el"
and load it in Emacs with M-x load-file
. Then start the game
with M-x minesweeper
.
Joe Marshall — Dependency Injection with Thunks vs. Global Variables
@2025-05-29 07:00 · 43 days agoRevision 2
A thunk (in MIT parlance) is a function that takes no arguments and returns a value. Thunks are simple, opaque objects. The only thing you can do with a thunk is call it. The only control you can exert over a thunk is whether and when to call it.
A thunk separates the two concerns of what to compute and when to compute it. When a thunk is created, it captures the lexical bindings of its free variables (it is, after all, just a lexical closure). When the thunk is invoked, it uses the captured lexical values to compute the answer.
There are a couple of common ways one might use a thunk. The first is to delay computation. The computation doesn't occur until the thunk is invoked. The second is as a weaker, safer form of a pointer. If the thunk is simply a reference to a lexical variable, then invoking the thunk returns the current value of the variable.
I once saw an article about Python that mentioned that you could create functions of no arguments. It went on to say that such a function had no use because you could always just pass its return value directly. I don't know how common this misconception is, but thunks are a very useful tool in programming.
Here is a use case that came up recently:
In most programs but the smallest, you will break the program into modules that you try to keep independent. The code within a module may be fairly tightly coupled, but you try to keep the dependencies between the modules at a minimum. You don't want, for example, the internals of the keyboard module to affect the internals of the display module. The point of modularizing the code is to reduce the combinatorics of interactions between the various parts of the program.
If you do this, you will find that your program has a “linking” phase at the beginning where you instantiate and initialize the various modules and let them know about each other. This is where you would use a technique like “depenedency injection” to set up the dependencies between the modules.
If you want to share data between modules, you have options. The crudest way to do this is to use global variables. If you're smart, one module will have the privilege of writing to the global variable and the other modules will only be allowed to read it. That way, you won't have two modules fighting over the value. But global variables come with a bit of baggage. Usually, they are globally writable, so nothing is enforcing the rule that only one module is in charge of the value. In addition, anyone can decide to depend on the value. Some junior programmer could add a line of code in the display handler that reads a global value that the keyboard module maintains and suddenly you have a dependency that you didn't plan on.
A better option is to use a thunk that returns the value you want to share. You can use dependency injection to pass the thunk to the modules that need it. When the module needs the value, it invokes the thunk. Modules cannot modify the shared value because the thunk has no way to modify what it returns. Modules cannot accidentally acquire a dependency on the value because they need the thunk to be passed to them explicitly upon initialization. The value that the thunk returns can be initialized after the thunk is created, so you can link up all the dependencies between the modules before you start computing any values.
I used this technique recently in some code. One thread sits in a loop and scrapes data from some web services. It updates a couple dozen variables and keeps them up to date every few hours. Other parts of the code need to read these values, but I didn't want to have a couple dozen global variables just hanging around flapping in the breeze. Instead, I created thunks for the values and injected them into the constructors for the URL handlers that needed them. When a handler gets a request, it invokes its thunks to get the latest values of the relevant variables. The values are private to the module that updates them, so no other modules can modify them, and they aren't global.
I showed this technique to one of our junior programmers the other day. He hadn't seen it before. He just assumed that there was a global variable. He couldn't figure out why the global variables were never updated. He was trying to pass global variables by value at initialization time. The variables are empty at that time, and updates to the variable later on have no effect on value that have already been passed. This vexed him for some time until I showed him that he should be passing a thunk that refers to the value rather than the value itself.
Joe Marshall — Vibe Coding Common Lisp Through the Back Door
@2025-05-28 07:00 · 44 days agoI had no luck vibe coding Common Lisp, but I'm pretty sure I know the reasons. First, Common Lisp doesn't have as much boilerplate as other languages. When boilerplace accumulates, you write a macro to make it go away. Second, Common Lisp is not as popular language as others, so there is far less training data.
Someone made the interesting suggestion of doing this in two steps: vibe code in a popular language, then ask the LLM to translate the result into Common Lisp. That sounded like it might work, so I decided to try it out.
Again, I used "Minesweeper" as the example. I asked the LLM to vibe code Minesweeper in Golang. Golang has a lot of boilerplate (it seems to be mostly boilerplate), and there is a good body of code written in Golang.
The first problem was that the code expected assets of images of the minesweeper tiles. I asked the LLM to generate them, but it wasn't keen on doing that. It would generate a large jpeg image of a field of tiles, but not a set of .png images of the tiles.
So I asked the LLM to vibe code a program that would generate the .png files for the tiles. It took a couple of iterations (the first time, the digits in the tiles were too small to read), but it eventually generated a program which would generate the tiles.
Then I vibe coded minesweeper. As per the philosophy of vibe coding, I did not bother writing tests, examining the code, or anything. I just ran the code.
Naturally it didn't work. It took me the entire day to debug this, but there were only two problems. The first was that the LLM simply could not get the API to the image library right. It kept thinking the image library was going to return an integer error code, but the latest api returns an Error interface. I could not get it to use this correctly; it kept trying to coerce it to an integer. Eventually I simply discarded any error message for that library and prayed it would work.
The second problem was vexing. I was presented with a blank screen. The game logic seemed to work because when I clicked around on the blank screen, stdout would eventually print "Boom!". But there were no visuals. I spent a lot of time trying to figure out what was going on, adding debugging code, and so on. I finally discovered that the SDL renderer was simply not working. It wouldn't render anything. I asked the LLM to help me debug this, and I went down a rabbit hole of updating the graphics drivers, reinstalling SDL, reinstalling Ubuntu, all to no avail. Eventually I tried using the SDL2 software renderer instead of the hardware accelerated renderer and suddenly I had graphics. It took me several hours to figure this out, and several hours to back out my changes tracking down this problem.
Once I got the tiles to render, though, it was a working Minesweeper game. It didn't have a timer and mine count, but it had a playing field and you could click on the tiles to reveal them. It had the look and feel of the real game. So you can vibe code golang.
The next task was to translate the golang to Common Lisp. It didn't do as good a job. It mentioned symbols that didn't exist in packages that didn't exist. I had to make a manual pass to replace the bogus symbols with the nearest real ones. It failed to generate working code that could load the tiles. I looked at the Common Lisp code and it was a horror. Not suprisingly, it was more or less a transliteration of the golang code. It took no advantage of any Common Lisp features such as unwind-protect. Basically, each and every branch in the main function had its own duplicate copy of the cleanup code. Since the tiles were not loading, I couldn't really test the game logic. I was in no mood to debug the tile loading (it was trying to call functions that did not exist), so I left it there.
This approach, vibe in golang and then translate to Common Lisp, seems more promising, but with two phase of LLM coding, the probability of a working result gets pretty low. And you don't really get Common Lisp, you get something closer to fully parenthesized golang.
I think I am done with this experiment for now. When I have some agentic LLM that can drive Emacs, I may try it again.
Joe Marshall — Thoughts on LLMs
@2025-05-27 14:55 · 45 days agoI've been exploring LLMs these past few weeks. There is a lot of hype and confusion about them, so I thought I'd share some of my thoughts.
LLMs are a significant step forward in machine learning. They have their limitations (you cannot Vibe Code in Common Lisp), but on the jobs they are good at, they are uncanny in their ability. They are not a replacement for anything, actually, but a new tool that can be used in many diverse and some unexpected ways.
It is clear to me that LLMs are the biggest thing to happen in computing since the web, and I don't say that lightly. I am encouraging everyone I know to learn about them, gain some experience with them, and learn their uses and limitations. Not knowing how to use an LLM will be like not knowing how to use a web browser.
Our company has invested in GitHub Copilot, which is nominally aimed at helping programmers write code, but if you poke around at it a bit, you can get access to the general LLM that underlies the code generation. Our company enables the GPT-4.1, Claude Sonnet 3.5, and Claude Sonnet 3.7 models. There is some rather complicated and confusing pricing for these models, and our general policy is to push people to use GPT-4.1 for the bulk of their work and to use Claude Sonnet models on an as-needed basis.
For my personal use, I decided to try a subscription to Google Gemini. The offering is confusing and I am not completely sure which services I get at which ancillary cost. It appears that there is a baseline model that costs nothing beyond what I pay for, but there are also more advanced models that have a per-query cost above and beyond my subscription. It does not help that there is a "1 month free trial", so I am not sure what I will eventually be billed for. (I am keeping an eye on the billing!) A subscription at around $20 per month seems reasonable to me, but I don't want to run up a few hundred queries at $1.50 each.
The Gemini offering appears to allow unlimited (or a large limit) of queries if you use the web UI, but the API incurs a cost per query. That is unfortunate because I want to use Gemini in Emacs.
Speaking of Emacs. There are a couple of bleeding edge Emacs packages that provide access to LLMs. I have hooked up code completion to Copilot, so I get completion suggestions as I type. I think I get these at zero extra cost. I also have Copilot Chat and Gemini Chat set up so I can converse with the LLM in a buffer. The Copilot chat uses an Org mode buffer whereas the Gemini chat uses a markdown buffer. I am trying to figure out how to hook up emacs as an LLM agent so that that the LLM can drive Emacs to accomplish tasks. (Obviously it is completely insane to do this, I don't trust it a bit, but I want to see the limitations.)
The Gemini offering also gives you access to Gemini integration with other Google tools, such as GMail and Google Docs. These look promising. There is also AIStudio in addition to the plain Gemini web UI. AIStudio is a more advanced interface that allows you to generate applications and media with the LLM.
I am curious about Groq. They are at a bit of a disadvantage in that they cannot integrate with Google Apps or Microsoft Apps as well as Gemini and Copilot can. I may try them out at some time in the future.
I encourage everyone to try out LLMs and gain some experience with them. I think we're on the exponential growth part of the curve at this point and we will see some amazing things in the next few years. I would not wait too long to get started. It will be a significant skill to develop.
vindarel — Hacker News now runs on top of Common Lisp
@2025-05-26 15:46 · 46 days agoHacker News was written in the Arc lisp dialect, a dialect created by Paul Graham. Arc was implemented on top of Racket, but that has now changed. HN runs on top of SBCL since (at least) September of 2024.
But why? For performance reasons.
I recently noticed that Hacker News no longer uses paging for long threads. In the past, when a discussion grew large, we had to click “More” to load the next page of comments, and dang would occasionally post helpful tips to remind us about this feature. Was there an announcement regarding this change? Has anyone else still seen paging recently? I’d love to know more details—especially the technical aspects or considerations that went into the decision.
Answer:
It’s because Clarc is finally out.
[Clarc] is much faster and also will easily let HN run on multiple cores. It’s been in the works for years, mainly because I rarely find time to work on it, but it’s all pretty close to done.
How it’s done:
there’s now an Arc-to-JS called Lilt, and an Arc-to-Common Lisp called Clarc. In order to make those easier to develop, we reworked the lower depths of the existing Arc implementation to build Arc up in stages. The bottom one is called arc0, then arc1 is written in arc0, and arc2 in arc1. The one at the top (arc2, I think) is full Arc. This isn’t novel, but it makes reimplementation easier since you pack as much as possible in the later stages, and only arc0 needs to be written in the underlying system (Racket, JS, or CL).
But Clarc’s code isn’t released, although it could be done:
open-sourcing the Arc implementation (i.e. Clarc) would be much easier [than the HN site]. The way to do it would be to port the original Arc release (http://arclanguage.org/) to Clarc. It includes a sample application which is an early version of HN, scrubbed of anything HN- or YC-specific.
Releasing the new HN code base however wouldn’t work:
Much of the HN codebase consists of anti-abuse measures that would stop working if people knew about them. Unfortunately. separating out the secret parts would by now be a lot of work. The time to do it will be if and when we eventually release the alternative Arc implementations we’ve been working on.
https://news.ycombinator.com/item?id=21546438
Congrats for the successful “splash-free” transition though.
- edit 10PM UTC+2: added a quote to clarify about open-sourcing the HN code (“wouldn’t work”) VS Clarc itself (“much easier”).
- edit May, 27th: reworded “since a few months” to mention a date.
Joe Marshall — Roll Your Own Bullshit
@2025-05-25 07:00 · 47 days agoMany people with pointless psychology degrees make money by creating corporate training courses. But you don't need a fancy degree to write your own training course. They all follow the same basic format:
- Pick any two axes of the Myers-Briggs personality test.
- Ask the participants to answer a few questions designed to determine where they fall on those axes. It is unimportant what the answers are, only that there is a distribution of answers.
- Since you have chosen two axes, the answers will, by necessity, fall into four quadrants. (Had you chosen three axes, you'd have eight octants, which is too messy to visualize.)
- Fudge the median scores so that the quadrants are roughly equal in size. If you have a lot of participants, you can use statistical methods to ensure that the quadrants are equal, but for small groups, just eyeball it.
- Give each quadrant a name, like “The Thinkers”, “The Feelers”, “The Doers”, and “The Dreamers”. It doesn't matter what you call them, as long as they sound good.
- Assign to each quadrant a set of traits that are supposed to be broad stereotypes of people in that quadrant. Again, it doesn't matter what you say, as long as it sounds good. Pick at least two positive and two negative traits for each quadrant.
- Assign each participant to a quadrant based on their answers.
- Have the participants break into focus groups for their quadrants and discuss among themselves how their quadrant relates to the other quadrants.
- Break for stale sandwiches and bad coffee.
- Have each group report back to the larger group, where they restate what they discussed in their focus groups.
- Conclude with a summary of the traits of each quadrant, and how they relate to each other. This is the most important part, because it is where you can make up any bullshit you want, and nobody will be able to call you on it. Try to sound as if you have made some profound insights.
- Have the participants fill out a survey to see how they feel about the training. This is important, because it allows you to claim that the training was a success.
- Hand out certificates of completion to all participants.
- Profit.
It is a simple formula, and it is apparently easy to sell such courses to companies. I have attended several of these courses, and they all follow this same basic formula. They are all a waste of time, and they are all a scam.
Joe Marshall — More Bullshit
@2025-05-24 15:24 · 48 days agoEarly on in my career at Google I attended an offsite seminar for grooming managers. We had the usual set of morning lectures, and then we were split into smaller groups for discussion. The topic was “What motivates people?” Various answers were suggested, but I came up with these four: Sex, drugs, power, and money.
For some reason, they were not happy with my answer.
They seemed to think that I was not taking the question seriously. But history has shown that these four things are extremely strong motivations. They can motivate people to do all sorts of things they wouldn't otherwise consider. Like, for example, to betray their country.
If you look for them, you can find the motivations I listed. They are thinly disguised, of course. Why are administrative assistants so often attractive young women? Is Google's massage program completely innocent? Are there never any “special favors”? The beer flows pretty freely on Fridays, and the company parties were legendary. Of course there are the stock options and the spot bonuses.
I guess I was being too frank for their taste. They were looking for some kind of “corporate culture” answer, like “team spirit” or “collaboration”. I was just being honest.
I soon discovered that the true topic of this three day offsite seminar was bullshit. It was a “course” dreamed up by business “psychologists” and peddled to Silicon Valley companies as a scam. If you've ever encountered “team building” courses, you'll know what I mean. It is a lucrative business. This was just an extra large helping.
They didn't like my frankness, and I didn't see how they could expect to really understand what motivates people if they were not going to address the obvious. They thought I wasn't being serious with my answer, but it was clear that they weren't going to be seriously examining the question either.
So I left the seminar. I didn't want to waste my time listening to corporate psychobabble. My time was better spent writing code and engineering software. I returned to my office to actually accomplish some meaningful work. No doubt I had ruined any chance of becoming a big-wig, but I simply could not stomach the phoniness and insincerity. I was not going to play that game.
Joe Marshall — Management = Bullshit
@2025-05-20 14:14 · 52 days agoThe more I have to deal with management, the more I have to deal with bullshit. The higher up in the management chain, the denser the bullshit. Now I'm not going to tell you that all management is useless, but there is a lot more problem generation than problem solving.
Lately I've been exploring the potentials of LLMs as a tool in my day-to-day work. They have a number of technical limitations, but some things they excel at. One of those things is generating the kinds of bullshit that management loves to wallow in. Case in point: our disaster recovery plan.
Someone in management got it into their head that we should have a formal disaster recovery plan. Certainly this is a good idea, but there are tradeoffs to be made. After all, we have yearly fire drills, but we don't practice "duck and cover" or evacuation in case of flooding. We have a plan for what to do in case of a fire, but we don't have a plan for what to do in case of a zombie apocalypse. But management wants a plan for everything, no matter how unlikely.
Enter the LLM. It can generate plans like nobody's business. It can generate a plan for what to do in case of a fire, a meteor strike, or a zombie apocalypse. The plans are useless, naturally. They are just bullshit. But they satisfy management's jonesing for plans, and best of all, they require no work on my part. It saved me hours of work yesterday.
Gábor Melis — PAX PDF Output
@2025-05-15 00:00 · 57 days agoThanks to Paul A. Patience,
PAX now has PDF support. See
pax-manual-v0.4.1.pdf and
dref-manual-v0.4.1.pdf. The PDF is very similar
to the HTML, even down to the locative types (e.g
[function]
) being linked to the sources on GitHub, but
cross-linking between PDFs doesn't work reliably on most viewers, so
that's disabled. Also, for reading PDFs so heavy on internal linking
to be enjoyable, one needs a viewer that supports going back within
the PDF (not the case with Chrome at the moment). Here is a blurry
screenshot to entice:
Joe Marshall — Purchasing White Elephants
@2025-05-13 20:05 · 59 days agoAs a software engineer, I'm constantly trying to persuade management to avoid doing stupid things. Management is of the opinion that because they are paying the engineers anyway, the software is essentially free. In my experience, bespoke software is one of the most expensive things you can waste money on. You're usually better off setting your money on fire than writing custom software.
But managers get ideas in their heads and it falls upon us engineers to puncture them. I wish I were less ethical. I'd just take the money and spend it as long as it kept flowing. But I wouldn't be able to live with myself. I have to at least try to persuade them to avoid the most egregious boondoggles. If they still insist on doing the project, well, so be it.
I'm absolutely delighted to find that these LLMs are very good at making plausible sounding proposals for software projects. I was asked about a project recently and I just fed the parameters into the LLM and asked it for an outline of the project, estimated headcount, time, and cost. It suggested we could do it in 6 months with 15 engineers at a cost of $3M. (I think it was more than a bit optimistic, frankly, but it was a good start.) It provided a phased breakdown of the project and the burn rate. Management was curious about how long it would take 1 engineer and the LLM suggested 3-6 years.
Management was suitably horrified.
I've been trying to persuade them that the status quo has been satisfying our needs, costs nothing, needs no engineers, and is ready today, but they didn't want to hear it. But now they are starting to see the light.
Marco Antoniotti — Getting into a rabbit's hole and - maybe - getting out: Emacs Make Compile (EMC)
@2025-05-12 13:41 · 60 days agoIn the past years I fell form one rabbit's hole into another. For the first time in a rather long time, I feel I am getting out of one.
First of all let me tell you what I produced. I built the "Emacs Make Compile",
or "Emacs Master of Ceremonies", package
EMC.
Soon it will be available in melpa.
The EMC package is a wrapper around compile.el
that allows you to semi-transparently
invoke make
or cmake
from Emacs. Either on UN*X, MacOS or Windows.
Once you have loaded the emc
library in the usual ways, you can just issue the
Emacs command emc:run
(yes: Common Lisp naming conventions). The command is just
the most general one available in EMC; other ones are the more specialized
emc:make
and emc:cmake
. Emacs will then ask you for the necessary
bits and pieces to ensure that you can run, say, make
.
The README file included in the distribution explains what is available in more details.
Where did it all begin?
Why not stick with compile.el
? Because it does not have "out-of-the-box" decent
defaults under Windows. At least, that was my original excuse.
I fell into this rabbit's hole coming from another one of course.
Some time ago, I started fiddling around with Emacs Dynamic Modules.
I wanted to compile them directly from Emacs in order to "simplify" their deployment. Therefore, I
set out to write a make
function that would hide the compile
setup.
Alas, I found out that, because of the necessary setup, invoking the
Microsoft Visual Studio toolchain
is not easy before you can get to cl
and nmake
. That was not all that difficult as a problem to solve, but then I
made the mistake of learning to cmake
. You know; to ensure that the building
process was "more portable". The basic machinery for make
and nmake
worked to also get cmake
up and running. But then I made another mistake: I
started to want to get EMC to be usable in the "Emacs" way: at a minimum getting
interactive commands working. That got me deeper and deeper in the rabbit's hole.
At the bottom of the hole (yep: I got there!)
I found out many things on my way to the bottom. That is, I learned many things about the Emacs Lisp ecosystem and wasted a lot of time in the process. I never was a fast learner. All in all, I think I can now say two things.
- Making a command, i.e., an
interactive
function is not trivial, especially if your function has many arguments. Bottom line: your Emacs commands should have *few* arguments. I should have known better. - The Emacs
widget
library is woefully underdocumented (which, of course, brings up the question: why did you want to use it?)
In any case, what I was able to concot is that hitting M-x emc:make
does what you
expect, assuming you have a Makefile
in the directory; if not you will be asked for
a "makefile", say stuff.mk
to be used as in
make -f stuff.mk
- or
nmake /F stuff.mk
Issuing C-u M-x emc:make
will ask you for the "makefile", the "source directory",
the "build directory", "macros", and "targets".
In what other ways could I have wasted some time? By coming up with a widget-based UI! (See my previous
post about DeepSeek and the widget library).
The result can be invoked by using the command emc:emc
, which pops up the
window below.
Getting out of the rabbit hole by popping the stack
I kind of consider EMC finished.
I am pleased by the result; it was fun to solve
all the problems I encountered, although the code is not exaclty nice or nicely organized.
Is EMC useful? Probabiy not so much, but I have the luxury
of wasting hacking time. I just hope somebody will like it: please try it out and report bugs and
suggestions (the minor mode and associated menu need work for sure, as well as emc:emc
).
Having said so, I can now go back to play with Emacs Dynamic Modules, which is where I was coming
from. After being satisfied with that, I will be able to climb back up a bit more from the rabbit's
hole; that is, I will be able to go back to the magiciel
library (which kind of works already). You may ask why
I am writing magiciel
, but you will have to reach down several levels in the rabbit's hole.
In any case, I finished one thing. It's progress.
'(cheers)
Gábor Melis — Adaptive Hashing
@2025-05-02 00:00 · 70 days agoAt the 2024 ELS, I gave a talk on adaptive hashing, which focusses on making general purpose hash tables faster and more robust at the same time.
Theory vs Practice
Hash table theory most concerns itself with the asymptotic worst-case cost with a hash function chosen randomly from a family of hash functions. Although these results are very relevant in practice,
those pesky constant factors, that the big-O cost ignores, do matter, and
we don't pick hash functions randomly but fix the hash function for the lifetime of the hash table.
There are Perfect Hashing algorithms, that choose an optimal hash function for a given set of keys. The drawback is that they either require the set of keys to be fixed or they are too slow to be used as general purpose hash tables.
Still, the idea that we can do better by adapting the hash function to the actual keys is key. Can we do that online, that is, while the hash table is being used? Potential performance gains come from improving the constant factors mentioned above by
having fewer collisions, and
being more cache-friendly.
The first image above plots the regret (the expected number of
comparisons of per lookup minus the minimum achievable) and the
measured run-time of PUT operations vs the number of keys in the
hash table with a particular key distribution. Green is Murmur (a
robust hash function), Blue is SBCL's expedient EQ
hash. The wiggling of the graphs is due to the resizing of the hash
table as keys are added to it.
Note how SBCL's regret starts out much lower and becomes much higher than that of Murmur, but if anything, its advantage in run time (second image) grows.
Implementation
The general idea is sound, but turning it into real performance gains is hard due to the cost of choosing a hash function and switching to it. First, we have to make some assumption about the distribution of keys. In fact, default hash functions in language runtimes often make such assumptions to make the common cases faster, usually at the cost of weakened worst-case guarantees.
The rest of this post is about how SBCL's built-in hash tables, which had been reasonably fast, were modified. The core switching mechanism looks at
the length of the collision chain on PUT operations,
the collision count on rehash (when the hash table is grown), and
the size of the hash table.
Adapting EQ
hash tables
Init to to constant hash function. This a fancy way of saying that we do linear search in a vector internally. This is an
EQ
hash table, so key comparison is as single assembly instruction.When the hash table is grown to more than 32 keys and it must be rehashed anyway, we switch to a hash function that does a single right shift with the number of bits to shift determined from the longest common run of low-bits in the keys.
If too many collisions, we switch to the previous default SBCL
EQ
-hash function that has been tuned for a long time.If too many collisions, we switch to Murmur, a general purpose hash. We could also go all the way to cryptographic hashes.
In step 2, the hash function with the single shift fits the memory allocator's behaviour nicely: it is a perfect hash for keys forming arithmetic sequences, which is often approximately true for objects of the same type allocated in a loop.
In this figure, the red line is the adaptive hash.
Adapting EQUAL
hash tables
For composite keys, running the hash function is the main cost. Adaptive hashing does the following.
For string keys, hash only the first and last 2 characters.
For list keys, only hash the first 4 elements.
If too many collisions, double the limit.
So, SBCL hash tables have been adaptive for almost a year now,
gaining some speed in common cases, and robustness in others.
The full paper is here.
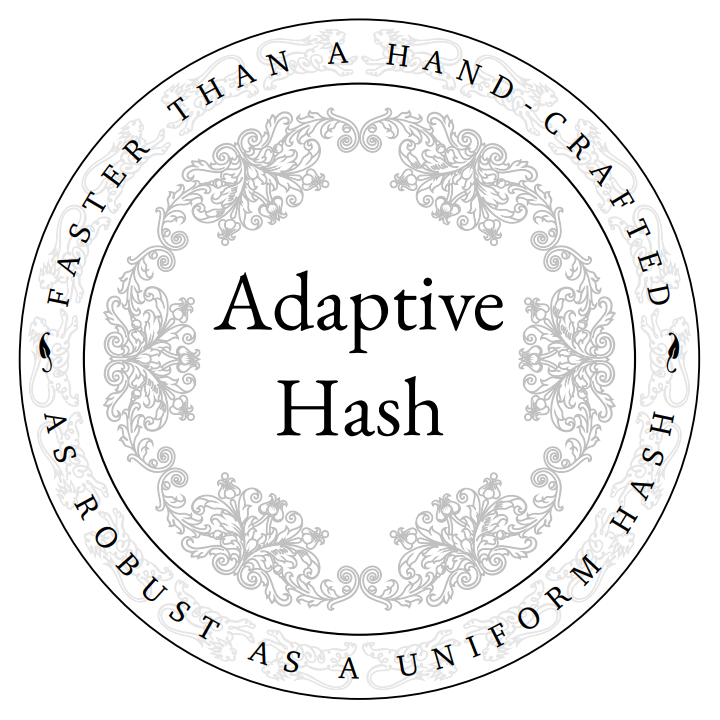
Joe Marshall — It Still Sucks
@2025-05-01 12:29 · 71 days agoDon’t get me wrong. I”m not saying that the alternatives are any better or even any different.
Unix has been around more than forty years and it is still susceptible to I/O deadlock when you try to run a subprocess and stream input to it and output from it. The processes run just fine for a while, then they hang indefinitely waiting for input and output from some buffer to synchronize.
I’m trying to store data in a database. There aren't any good database bindings I could find, so I wrote a small program that reads a record from stdin and writes it to the database. I launch this program from Common Lisp and write records to the input of the program. It works for about twenty records and then hangs. I've tried to be careful to flush and drain all streams from both ends, to no avail.
I have a workaround: start the program, write one record, and quit the program. This doesn’t hang and reliably writes a record to the database, but it isn’t fast and it is constantly initializing and creating a database connection and tearing it back down for each record.
You'd think that subprocesses communicating via stream of characters would be simple.
Neil Munro — Ningle Tutorial 6: Database Connections
@2025-04-30 21:30 · 71 days agoContents
- Part 1 (Hello World)
- Part 2 (Basic Templates)
- Part 3 (Introduction to middleware and Static File management)
- Part 4 (Forms)
- Part 5 (Environmental Variables)
- Part 6 (Database Connections)
- Part 7 (Envy Configuation Switching)
- Part 8 (Mouning Middleware)
Introduction
Welcome back, in this tutorial we will begin looking at how to work with SQL databases, specifically SQLite3, MySQL, and PostgreSQL. We will be using the mito ORM to create user models and save them to the database using the form
we created previously. Mito
itself is a basic ORM and includes several mixins to provide additional functionality, we will use one called mito-auth to provide password hashing and salting.
It is important to know that mito
is based on top of a SQL library known as SXQL, we will occasionally use SXQL
to write queries with mito
, while it's not always required to use SXQL, there are times where it will make life easier. To achieve this, I elected to :use
SXQL in my package definition.
(defpackage ningle-tutorial-project
(:use :cl :sxql)
Part of working with databases using an ORM is creating the initial database/tables and managing changes over time, called migrations
, mito
appears to have a migrations system, although I was unable to get it working, but I developed a means by which to apply migrations, so perhaps in a future tutorial the subject can be revisited. As such, in addition to seeing how to connect to the respective SQL databases, we will write implementation specific migration functions.
We will follow the example of setting up a secure user registration system across all three SQL implementations. One thing to bear in mind is that it is beyond the scope of this tutorial to instruct how to setup MySQL or PostgreSQL, I would recommend learning how to set them up using docker. All that having been said, let's have a look at the different databases and how to connect to them!
Please bear in mind that when working with SQLite remember to add .db
to your .gitignore
as you most certainly don't want to accidentally commit a database into git! SQLite, being a file based database (unlike MySQL and PostgreSQL) will create a file that represents your database so this step only applies to SQLite.
Installing Packages
To begin with we will need to ensure we have installed and added the packages we need to our project asd file, there are three that we will be installing:
As normal you will need to add them in the :depends-on
section. Please note however that there is an issue in mito-auth
that prevents it from working in MySQL, I have submitted a fix but it has not been merged yet, so for now you can use my branch, if you do, please ensure you check it out via git into your quicklisp/local-projects
directory.
:depends-on (:clack
:cl-dotenv
:djula
:cl-forms
:cl-forms.djula
:cl-forms.ningle
:ingle ;; Add this
:mito ;; Add this
:mito-auth ;; Add this
:ningle)
Mito is a package for managing models/tables in our application, mito-auth is a mixin
that enables models to have a secure password field, not all models will need this, but our user model will! ingle
is a small library that includes some very useful utilities, one of which is a redirect
function which will be very useful indeed!
Now that that is done, we must set up the middleware, you might remember from Part 3 that middleware is placed in the lack.builder:builder
function call in our start
function.
SQL Middleware
Mito provides middleware to establish and manage database connections for SQLite3
, MySQL
, and PostgreSQL
, when you build your solution you will need to pick a database implementation, for production systems I suggest PostgreSQL
, but if you are just starting out, you can use SQLite
.
SQLite3
(defun start (&key (server :woo) (address "127.0.0.1") (port 8000))
(djula:add-template-directory (asdf:system-relative-pathname :ningle-tutorial-project "src/templates/"))
(djula:set-static-url "/public/")
(clack:clackup
(lack.builder:builder
:session
`(:mito
(:sqlite3
:database-name ,(uiop:getenv "SQLITE_DB_NAME")))
(:static
:root (asdf:system-relative-pathname :ningle-tutorial-project "src/static/")
:path "/public/")
*app*)
:server server
:address address
:port port))
MySQL
(defun start (&key (server :woo) (address "127.0.0.1") (port 8000))
(djula:add-template-directory (asdf:system-relative-pathname :ningle-tutorial-project "src/templates/"))
(djula:set-static-url "/public/")
(clack:clackup
(lack.builder:builder
:session
`(:mito
(:mysql
:database-name ,(uiop:native-namestring (uiop:parse-unix-namestring (uiop:getenv "MYSQL_DB_NAME")))
:username ,(uiop:getenv "MYSQL_USER")
:password ,(uiop:getenv "MYSQL_PASSWORD")
:host ,(uiop:getenv "MYSQL_ADDRESS")
:port ,(parse-integer (uiop:getenv "MYSQL_PORT"))))
(:static
:root (asdf:system-relative-pathname :ningle-tutorial-project "src/static/")
:path "/public/")
*app*)
:server server
:address address
:port port))
PostgreSQL
(defun start (&key (server :woo) (address "127.0.0.1") (port 8000))
(djula:add-template-directory (asdf:system-relative-pathname :ningle-tutorial-project "src/templates/"))
(djula:set-static-url "/public/")
(clack:clackup
(lack.builder:builder
:session
`(:mito
(:postgres
:database-name ,(uiop:native-namestring (uiop:parse-unix-namestring (uiop:getenv "POSTGRES_DB_NAME")))
:username ,(uiop:getenv "POSTGRES_USER")
:password ,(uiop:getenv "POSTGRES_PASSWORD")
:host ,(uiop:getenv "POSTGRES_ADDRESS")
:port ,(parse-integer (uiop:getenv "POSTGRES_PORT"))))
(:static
:root (asdf:system-relative-pathname :ningle-tutorial-project "src/static/")
:path "/public/")
*app*)
:server server
:address address
:port port))
Testing The Connection
Before we go further with building models and migration functions, we should test that the connections work and the most basic of SQL statements. We will be working on our register controller, so that seems like as good a place as any to place a simple check.
(setf (ningle:route *app* "/register" :method '(:GET :POST))
(lambda (params)
(let ((query (mito:retrieve-by-sql "SELECT 2 + 3 AS result")))
(format t "Test: ~A~%" query))
(let ((form (cl-forms:find-form 'register)))
...
Here, in the controller we have added a small (temporary) check to ensure that the database connections are set up correctly, when you run the application and perform a GET
request on this route, you should see the output printed in the console for:
Test: ((RESULT 5))
It might look a little odd, but rest assured that this is proof that everything is right and the connection works! We will be removing this later as it serves just as a small check. So with that done, we can begin to look into writing our first model, our user model.
Creating Models
Models are a way to represent both a generic object, and any specific object of that type in a relational database system. For example you might have a Book model, that represents a book table, however a book is just a way to classify something any doesn't tell you anything specific about any individual book. So here we will create a User model, that refers to all users, but each instance of a User will contain the specific information about any given user.
We will create a new file called models.lisp
:
(defpackage ningle-tutorial-project/models
(:use :cl :mito)
(:export #:user))
(in-package ningle-tutorial-project/models)
(deftable user (mito-auth:has-secure-password)
((email :col-type (:varchar 255) :initarg :email :accessor email)
(username :col-type (:varchar 255) :initarg :username :accessor username))
(:unique-keys email username))
Now, mito
provides a deftable
macro
that hides some of the complexities, there is a way to use a regular class and change the metaclass
, but it's much less typing and makes the code look nicer to use the deftable
syntax. It is important to note however that we use the mixin
from mito-auth
called has-secure-password
. Obviously this mixin wouldn't be needed in all of our models, but because we are creating a user that will log into our system, we need to ensure we are handling passwords securely.
Writing Migrations
Now that we have this we need to write the migration code I mentioned earlier, databases (and their models) change over time as application requirements change, as columns get added, removed, changed, etc it can be tricky to get right and you certainly would prefer to have these done automatically, a stray SQL query in the wrong connected database can do incredible damage (trust me, I know!), so migrations allow us to track these changes and have the database system manage them for us.
This code will set up connections to the implementation we want to use and delegate migrations to mito, so pick your implementation and place it in migrations.lisp
.
SQLite3
(defpackage ningle-tutorial-project/migrations
(:use :cl :mito)
(:export #:migrate))
(in-package :ningle-tutorial-project/migrations)
(defun migrate ()
"Explicitly apply migrations when called."
(dotenv:load-env (asdf:system-relative-pathname :ningle-tutorial-project ".env"))
(format t "Applying migrations...~%")
(mito:connect-toplevel
:sqlite3
:database-name (uiop:native-namestring (uiop:parse-unix-namestring (uiop:getenv "SQLITE_DB_NAME"))))
(mito:ensure-table-exists 'ningle-tutorial-project/models:user)
(mito:migrate-table 'ningle-tutorial-project/models:user)
(mito:disconnect-toplevel)
(format t "Migrations complete.~%"))
MySql
(defpackage ningle-tutorial-project/migrations
(:use :cl :mito)
(:export #:migrate))
(in-package :ningle-tutorial-project/migrations)
(defun migrate ()
"Explicitly apply migrations when called."
(dotenv:load-env (asdf:system-relative-pathname :ningle-tutorial-project ".env"))
(format t "Applying migrations...~%")
(mito:connect-toplevel
:mysql
:database-name (uiop:native-namestring (uiop:parse-unix-namestring (uiop:getenv "MYSQL_DB_NAME")))
:username (uiop:getenv "MYSQL_USER")
:password (uiop:getenv "MYSQL_PASSWORD")
:host (uiop:getenv "MYSQL_ADDRESS")
:port (parse-integer (uiop:getenv "MYSQL_PORT")))
(mito:ensure-table-exists 'ningle-tutorial-project/models:user)
(mito:migrate-table 'ningle-tutorial-project/models:user)
(mito:disconnect-toplevel)
(format t "Migrations complete.~%"))
PostgreSQL
(defpackage ningle-tutorial-project/migrations
(:use :cl :mito)
(:export #:migrate))
(in-package :ningle-tutorial-project/migrations)
(defun migrate ()
"Explicitly apply migrations when called."
(dotenv:load-env (asdf:system-relative-pathname :ningle-tutorial-project ".env"))
(format t "Applying migrations...~%")
(mito:connect-toplevel
:postgres
:database-name (uiop:getenv "POSTGRES_DB_NAME")
:host (uiop:getenv "POSTGRES_ADDRESS")
:port (parse-integer (uiop:getenv "POSTGRES_PORT"))
:username (uiop:getenv "POSTGRES_USER")
:password (uiop:getenv "POSTGRES_PASSWORD"))
(mito:ensure-table-exists 'ningle-tutorial-project/models:user)
(mito:migrate-table 'ningle-tutorial-project/models:user)
(mito:disconnect-toplevel)
(format t "Migrations complete.~%"))
It will be necessary to add these two files into the :components
section of your project asd file.
:components ((:module "src"
:components
((:file "models")
(:file "migrations")
(:file "forms")
(:file "main"))))
Just remember if you are using MySQL or PostgreSQL, you will need to ensure that the database you want to connect to exists (in our case ntp), and that your connecting user has the correct permissions!
Running Migrations
Now that everything is set up, we will need to perform our initial migrations:
(ningle-tutorial-project/migrations:migrate)
If this has worked, you will see a lot of output SQL statements, it's quite verbose, however this only means that it is working and we can move onto actually creating and saving models.
Removing Connection Check
Now that we have migrations and models working we should remember to remove this verification code that we wrote earlier.
(let ((query (mito:retrieve-by-sql "SELECT 2 + 3 AS result")))
(format t "Test: ~A~%" query))
Registering & Querying Users
What we are going to do now is use the user register form and connect it to our database, because we are registering users we will have to do some checks to ensure since we stated that usernames and email addresses are unique, we might want to raise an error.
(when valid
(cl-forms:with-form-field-values (email username password password-verify) form
(when (mito:select-dao 'ningle-tutorial-project/models:user
(where (:or (:= :username username)
(:= :email email))))
(error "Either username or email is already registered"))
We can see from this snippet here that mito uses the SXQL Domain Specific Language for expressing SQL statements. Using the select-dao
we can query the user table and apply where
clauses using a more Lispy like syntax to check to see if an account with the username or email already exists. Such DSLs are common when interacting with SQL inside another programming language, but it's good to know that from what we learned earlier that it can handle arbitrary SQL strings or this more Lispy syntax, so you can use pure SQL syntax, if necessary.
While having this check isn't necessary, it does make the error handling somewhat nicer, as well as exploring parts of the mito api. We will also add a check to raise an error if the passwords submitted in the form do not match each other.
(when (string/= password password-verify)
(error "Passwords do not match"))
If both of these pass (and you can test different permutations of course), we can continue to using mito to create our first user object!
(mito:create-dao 'ningle-tutorial-project/models:user
:email email
:username username
:password password)
The final thing to add is that we should redirect to another route, which we can do with the ingle:redirect
function.
(ingle:redirect "/people")
You will notice that we are redirecting to a route that doesn't (yet) exist, we will write the controller below after we have finished this controller, the multiple-value-bind
section of which, when completed, looks like this:
(multiple-value-bind (valid errors)
(cl-forms:validate-form form)
(when errors
(format t "Errors: ~A~%" errors))
(when valid
(cl-forms:with-form-field-values (email username password password-verify) form
(when (mito:select-dao 'ningle-tutorial-project/models:user
(where (:or (:= :username username)
(:= :email email))))
(error "Either username or email is already registered"))
(when (string/= password password-verify)
(error "Passwords do not match"))
(mito:create-dao 'ningle-tutorial-project/models:user
:email email
:username username
:password password)
(ingle:redirect "/people"))))
Getting All Users
We will look at two final examples of using mito before we finish this tutoral, as mentioned earlier we will write a new /people
controller, which will list all the users registered in the system, and we will create a /people/:person
controller to show the details of an individual user.
Starting with the /people
controller, we create a controller like we have seen before, we then use a let
binding to store the result of (mito:retrieve-dao 'ningle-tutoral-project/model:user)
, this is how we would get all rows from a table represented by the class 'ningle-tutorial-project/models:user
. We then pass the results into a template.
(setf (ningle:route *app* "/people")
(lambda (params)
(let ((users (mito:retrieve-dao 'ningle-tutorial-project/models:user)))
(djula:render-template* "people.html" nil :title "People" :users users))))
The html for this is written as such:
{% extends "base.html" %}
{% block content %}
<div class="container">
<div class="row" >
<div class="col-12">
{% for user in users %}
<div class="row mb-4">
<div class="col">
<div class="card">
<div class="card-body">
<h5 class="card-title"><a href="/people/{{ user.username }}">{{ user.username }}</a></h5>
<p class="card-text"><a href="/people/{{ user.email }}">{{ user.email }}</a></p>
<p class="text-muted small"></p>
</div>
</div>
</div>
</div>
{% endfor %}
{% if not users %}
<div class="row">
<div class="col text-center">
<p class="text-muted">No users to display.</p>
</div>
</div>
{% endif %}
</div>
</div>
</div>
{% endblock %}
Getting A Single User
In our individual person view, we see how a route may have variable data, our :person
component of the URL string, this will be either a username or email, it doesn't really matter which as we can have a SQL query that will find a record that will match the :person
string with either the username or email. We also take advantage of another ingle
function, the get-param
, which will get the value out of :person
. We use a let*
binding to store the user
derived from :person
and the result of mito:select-dao
(using the person
), we then pass the user
object into a template.
As we saw before this query was used to check for the existence of a username or email address in our register
controller.
(setf (ningle:route *app* "/people/:person")
(lambda (params)
(let* ((person (ingle:get-param :person params))
(user (first (mito:select-dao
'ningle-tutorial-project/models:user
(where (:or (:= :username person)
(:= :email person)))))))
(djula:render-template* "person.html" nil :title "Person" :user user))))
And here is the template for an individual user.
{% extends "base.html" %}
{% block content %}
<div class="container">
<div class="row">
<div class="col-12">
<div class="row mb-4">
<div class="col">
{% if not user %}
<h1>No users</h1>
{% else %}
<div class="card">
<div class="card-body">
<h5 class="card-title">{{ user.username }}</h5>
<p class="card-text">{{ user.email }}</p>
<p class="text-muted small"></p>
</div>
</div>
{% endif %}
</div>
</div>
</div>
</div>
</div>
{% endblock %}
Conclusion
This was a rather large chapter and we covered a lot, looking at the different means by which to connect to a SQL database, defining models, running migrations and executing queries, of course we are just getting started but this is a massive step forward and our application is beginning to take shape. I certainly hope you have enjoyed it and found it useful!
To recap, after working your way though this tutorial you should be able to:
- Explain what a model is
- Explain what a migration is
- Write code to connect to a SQL database
- Implement a model
- Implement a migration
- Execute a migration
- Write controllers that write information to a database via a model
- Write controllers that read information from a database via a model
Github
- The link for the SQLite version of this tutorial code is available here.
- The link for the MySQL version of this tutorial code is available here.
- The link for the PostgreSQL version of this tutorial code is available here.
Resources
Joe Marshall — Senior Programmers Have Nothing to Fear From AI
@2025-04-27 14:33 · 75 days agoI have, through experimentation, discovered that vibe coding in Common Lisp is not effective. But other kinds of AI coding are highly effective and have been saving me hours of work. AI is not going to replace senior programmers, but it will take over many of the tasks that junior programmers do. I’m not worried about my job, but were I a junior programmer, I’d be concerned.
Part of my job as a senior programmer is to identify tasks that are suitable for junior programmers. These tasks have certain properties:
- They are well-defined.
- They are repetitive, making them suitable for development of a program to carry them out.
- They are not too difficult, so that a junior programmer can complete them with a little help.
- They have a clear acceptance criteria, so that the junior programmer can tell when they are done.
- They have a clear abstraction boundary so that integrating the code after the junior programmer is done is not too difficult.
But because junior programmers are human, we have to consider these factors as well:
- The task must not be too simple or too boring.
- The task must be written in a popular programming language. Junior programmers don’t have the inclination to learn new programming languages.
- The task must not be time critical because junior programmers are slow.
- The task should not be core critical to the larger project. Junior programmers write crappy code, and you don’t want crappy code at the heart of your project.
Oftentimes, I find some tasks that fits many of these criteria, but then I find that I can do it myself better and faster than a junior programer could.
AI coding can handle many of the tasks that I would otherwise assign to a junior programmer. It works best when the task is well defined, not too difficult, and written in a popular language. It doesn’t care if the task is boring and repetitive. AI coding is much faster than a junior programmer, and it writes code that tends to follow standard conventions. If you can specify good abstraction barriers, the AI can do a good job of coding to them. While AI coding is not perfect, neither are junior programmers. In either case, a senior programmer needs to carefully review the code.
AI coding is not going to replace senior programmers. The AI will not generate code without a prompt, and the better the prompt, the better the generated code. Senior programmers can take a large program and break it down into smaller tasks. They can create definitions of the smaller tasks and define the acceptance criteria, the API, and the abstractions to be used. They can carefully and precisely craft the prompts that generate the code. Senior programmers are needed to drive the AI.
Which leads to the question of where senior programmers will come from if junior programmers are no longer needed. I don’t have a good answer for this.
Nicolas Martyanoff — Working with Common Lisp pathnames
@2025-04-26 18:00 · 76 days agoCommon Lisp pathnames, used to represent file paths, have the reputation of being hard to work with. This article aims to change this unfair reputation while highlighting the occasional quirks along the way.
Filenames and file paths
The distinction between filename and file paths is not always obvious. On POSIX systems, the filename is the name of the file, while a file path represents its absolute or relative location in the file system. Which also means that all filenames are file paths, but not the other way around.
Common Lisp uses the term filename for objects which are either pathnames or namestrings, both being representation of file paths. We will try to avoid confusion by using the terms filenames, pathnames and namestrings when referring to Common Lisp concepts and we will talk about file paths when referring to the language-agnostic file system concept.
Pathnames
Pathnames are an implementation-independent representation of file paths made of six components:
- an host identifying either the file system or a logical host;
- a device identifying the logical of physical device containing the file;
- a directory representing an absolute or relative list of directory names;
- a name;
- a type, a value nowadays known as file extension;
- a version, because yes file systems used to support file versioning.
While this representation might seem way too complicated —it originates from a time where the file system ecosystem was much richer— it still is suitable for modern file systems.
The make-pathname
function is used to create pathnames and lets you specificy
all components. For example the following call yields a pathname representing
the file path represented on POSIX systems by /var/run/example.pid
:
(make-pathname :directory '(:absolute "var" "run") :name "example" :type "pid")
Common Lisp functions manipulating file paths of course accept pathnames, letting you keep the same convenient structured representation everywhere, only converting from/to a native representation at the boundaries of your program.
Special characters
What happens when you construct a pathname with components containing separation
characters, e.g. a directory name containing /
on a POSIX system or a type
containing .
? According to Common Lisp 19.2.2.1.1, the behaviour is
implementation-defined; but if the implementation accepts these component values
it must handle quoting correctly.
For example:
- CLISP rejects separator characters in component values, signaling a
SIMPLE-ERROR
condition. - CCL accepts them and quotes them when converting the pathname to a
namestring. So
(namestring (make-pathname :name "foo/bar" :type "a.b"))
yields"foo\\/bar.a\\.b"
on Linux. - SBCL accepts and quotes them but does not quote
.
in type components, yielding"foo\\/bar.a.b"
for the example above. - ECL accepts them but fails to quote them when converting the pathname to a namestring.
One could wonder about which implementation, CCL or SBCL, is correct regarding
the quoting of the .
character in type strings on POSIX platforms. While
everyone understands that /
is special in file and directory names, .
is
debatable because POSIX does not mention the type extension in its definitions:
foo.txt
is the name of the file, not a combination of a name and a type. As
such, I would argue that quoting and not quoting are both correct. And as you
will realize then reading about namestrings further in this article, it is
irrelevant since namestrings are not POSIX paths.
Note that whether ECL violates the standard or not is unclear since there is no
character quoting for POSIX paths. In other words, there is no such thing as a
directory named a/b
, because it could not be referenced in a way different
from a directory named b
in a directory named a
. This behaviour derives
directly from POSIX systems treating paths as strings and not as structured
objects.
Invalid characters
The Common Lisp standard mentions special characters but is silent on the subject of invalid characters. For example POSIX forbids null bytes in filenames. But since it is not a separation character, implementations are free to deal with it as they see fit.
When testing implementations with a pathname containing a null byte using
(make-pathname :name (string (code-char 0)))
, CCL, SBCL and ECL accept it
while CLISP signals an error mentioning an illegal argument.
I am not convinced by CLISP’s behaviour since null bytes are only invalid in POSIX paths, not in Common Lisp filenames, meaning that the error should occur when the pathname is internally converted to a format usable by the operating system.
Pathname component case
A rarely mentioned property of pathnames is the support for case conversion.
MAKE-PATHNAME
and function returning pathname components (e.g.
PATHNAME-TYPE
) support a :CASE
argument, either :COMMON
or :LOCAL
indicating how how to handle character case in strings.
With :LOCAL
—which is the default value—, these functions assume that
component strings are already represented following the conventions of the
underlying operating system. It also dictates that if the host only supports one
character case, strings must be returned converted to this case.
With :COMMON
, these functions will use the default (customary) case of the
host if the string is provided all uppercase, and the opposite case if the
string is provided all lowercase. Mixed case strings are not transformed.
These behaviours are not intuitive and made much more sense at a time where some file systems only supported one specific case. You should probably stay away from component case handling unless you really know what you are doing.
On a personal note, as someone running Linux and FreeBSD, I am curious about the behaviour of various implementations on Windows and MacOS since both NTFS and APFS are case insensitive.
Unspecific components
While all components can be null, some of them can be :UNSPECIFIC
(which ones
is implementation-defined). The only real use case for :UNSPECIFIC
is to
affect the behaviour of MERGE-PATHNAMES
: if a component is null, the function
uses the value of the component in the pathname passed as the :DEFAULTS
argument; if a component is :UNSPECIFIC
, the function uses the same value in
the resulting pathname.
For example:
(merge-pathnames (make-pathname :name "foo")
(make-pathname :type "txt"))
yields the "foo.txt"
namestring, but
(merge-pathnames (make-pathname :name "foo" :type :unspecific)
(make-pathname :type "txt"))
yields "foo"
.
Unfortunately the inability to rely on its support for specific component types (since it is implementation-defined) makes it interesting more than useful.
Namestrings
Namestrings are another represention for file paths. While pathnames are
structured objects, namestrings are just strings. The most important aspect of
namestrings is that unless they are logical namestrings (something we will cover
later), the way they represent paths is implementation-defined (c.f. Common Lisp
19.1.1 Namestrings as Filenames). In other words the namestring for the file
foo
of type txt
in directory data
could be data/foo.txt
. Or
data\foo.txt
. Or data|foo#txt
. Or any other non-sensical representation.
Fortunately implementations tend to act rationally and use a representation as
similar as possible to the one of their host operating system.
One should always remember that even though namestrings look and feel like
paths, they are still a representation of a Common Lisp pathname, meaning that
they may or may not map to a valid native path. The most obvious example would
be a pathname whose name is the null byte, created with (make-pathname :name (string (code-char 0)))
, whose namestring is a one character string that has no
valid native representation on modern operating systems.
Pathnames can be converted to namestrings using the NAMESTRING
function, while
namestrings can be parsed into pathnames with PARSE-NAMESTRING
. The #P
reader macro uses PARSE-NAMESTRING
to read a pathname. As such,
#P"/tmp/foo.txt"
is identical to #.(parse-namestring '"/tmp/foo.txt")
.
Note that most functions dealing with files will accept a pathname designator, i.e. either a pathname, a namestring or a stream.
Native namestrings
An unfortunately missing feature from Common Lisp is the ability to parse native namestrings, i.e. paths that use the representation of the underlying operating system.
To understand why it is a problem, let us take *.txt
, a perfectly valid
filename at least on any POSIX platform. In Common Lisp, you can construct a
pathname representing this filename with (make-pathname :name "*" :type "txt")
. No problem whatsoever. However the "*.txt"
namestring actually
represents a pathname whose name component is :WILD
. There is no namestring
that will return this pathname when passed to PARSE-NAMESTRING
.
As a result, when processing filenames coming from the external world (a command line argument, a list of paths in a document, etc.), you have no way to handle those that contain characters used by Common Lisp for wild components.
There is no standard way of solving this issue. Some implementations provide
functions to parse native namestrings, e.g. SBCL with
SB-EXT:PARSE-NATIVE-NAMESTRING
or CCL with CCL:NATIVE-TO-PATHNAME
. If you
use ASDF, you can also use
UIOP:PARSE-NATIVE-NAMESTRING
.
Wildcards
Up to now pathnames may have looked like a slightly unusual representation for paths. But we are just getting started.
Pathname can be wild, meaning that they contain one or more wild components.
Wild components can match any value. All components can be made wild with the
special value :WILD
. Directory elements also support :WILD-INFERIORS
which
matches one or more directory levels.
As such
(make-pathname :directory '(:absolute "tmp" :wild) :name "foo" :type :wild)
is equivalent to the /tmp/*/foo.*
POSIX glob pattern, while
(make-pathname :directory '(:absolute "tmp" :wild-inferiors "data" :wild)
:name :wild :type :wild)
is equivalent to /tmp/**/data/*/*.*
.
Wild pathnames only really make sense for the DIRECTORY
function which returns
files matching a specific pathname.
Logical pathnames
We currently have talked about pathnames representing either paths to physical files or pattern of filenames. Logical pathnames go further and let you work with files in a location-independent way.
Logical pathnames are based on logical hosts, set as pathname host components. Logical pathnames can be passed around and manipulated as any other pathnames; when used to access files, they are translated to a physical pathname, i.e. a pathname referring to an actual file in the file system.
SBCL uses logical pathnames for source file locations. While SBCL is shipped
with its source files, their actual location on disk depends on how the software
was installed. Instead of manually merging pathnames with a base directory value
everywhere, SBCL uses the SYS
logical host to map all pathnames whose
directory starts with SRC
to the actual location on disk. For example on my
machine:
(translate-logical-pathname "SYS:SRC;ASSEMBLY;MASTER.LISP")
yields #P"/usr/share/sbcl-source/src/assembly/master.lisp"
.
Another example would be CCL which maps pathnames with the HOME
logical host
to subpaths of the home directory of the user.
Note that logical hosts are global to the Common Lisp environment. While SYS
is reserved for the implementation, all other hosts are free to use by anyone.
To avoid collisions, it is a good idea to name hosts after their program or
library.
Logical namestrings
Logical namestrings are implementation-independent, meaning that you can safely use them in your programs without wondering about how they will be interpreted. Their syntax, detailed in section 19.3.1 of the Common Lisp standard, is quite different from usual POSIX paths. The host is separated from the rest of the path by a colon character, and directory names are separated by semicolon characters.
For example "SOURCE:SERVER;LISTENER.LISP"
is the logical namestring equivalent
of the /server/listener.lisp
POSIX path for the SOURCE
logical host.
The astute reader will notice the use of uppercase characters in logical namestrings. It happens that the different parts of a logical namestring are defined as using uppercase characters, but that the implementation translates lowercase letters to uppercase letters when parsing the namestrings (c.f. Common Lisp 19.3.1.1.7). We use the canonical uppercase representation for clarity.
Translation
Translation is controlled by a table that maps logical hosts to a list of pattern (wild pathnames or namestrings) and their associated wild physical pathnames.
One can obtain the list of translations for a logical host with
LOGICAL-PATHNAME-TRANSLATIONS
and update it with (SETF LOGICAL-PATHNAME-TRANSLATIONS)
. Each translation is a list where the first
element is a logical pathname or namestring (usually a wild pathname) and the
second element is a pathname or namestring to translate into.
The translation process looks for the first entry that satisfies
PATHNAME-MATCH-P
, which is guaranteed to behave in a way consistent with
DIRECTORY
. When there is match, the translation processes replaces
corresponding patterns for each components.
And of course if translation results in a logical pathname, it will be recursively translated until a physical pathname is obtained.
A simple example would be the use of a logical host referring to a temporary directory. This lets a program manipulates temporary pathnames without having to know their actual physical location, the translation process being controlled in a single location.
(setf (logical-pathname-translations "tmp")
(list (list (make-pathname :host "tmp"
:directory '(:absolute :wild-inferiors)
:name :wild :type :wild)
(make-pathname :directory '(:absolute "var" "tmp" :wild-inferiors)
:name :wild :type :wild))))
or if we were to use namestrings:
(setf (logical-pathname-translations "tmp")
'(("TMP:**;*.*.*" "/var/tmp/**/*.*")))
Translating pathnames or namestrings using the TMP
logical host yields the
expected results. For example (translate-logical-pathname "TMP:CACHE;DATA.TXT")
yields #P"/var/tmp/cache/data.txt"
.
Caveats
While logical pathnames are an elegant abstraction, they are plagued by multiple issues that make them hard to use correctly and in a portable way.
Logical namestring components can only contain letters, digits and hyphens (or
the *
and **
sequences for wild namestrings). This limitation probably comes
from a need to be compatible with all existing file systems, but it can be a
showstopper if one needs to refer to files whose naming scheme is not controlled
by the program.
Namestring parsing is confusing: calling PARSE-NAMESTRING
on an invalid
namestring (because it contains invalid characters or because the host is not a
known logical host) will not fail. Instead the string will be parsed as a
physical namestring, introducing silent bugs. The LOGICAL-PATHNAME
can be used
to validate logical pathnames and namestrings.
The way translation converts between both pathname patterns is unclear. It is not specified by the Common Lisp standard. Debugging patterns can quickly become very frustrating, especially with implementations unable to produce quality error diagnostics.
Finally, the behaviour of logical pathnames with other functions is rarely obvious, leading to frustrating debugging sessions.
They nevertheless are a unique and helpful feature for very specific use cases.
Recipes
Resolving a path
Files are accessible through multiple paths. For example, on POSIX systems,
foo/bar/baz.txt
, foo/bar/../bar/baz.txt
refer to the same file. If your
operating system and file system support symbolic links, you can refer to the
same physical file from multiple links, themselves being files.
It is sometimes useful to obtain the canonical path of a file. On POSIX systems,
the realpath
function serves this purpose. In Common Lisp, this canonical path
is called truename, and the TRUENAME
function returns it.
Transforming paths
The :DEFAULTS
option of MAKE-PATHNAME
is useful to construct a pathname that
is a variation of another pathname. When a component passed to MAKE-PATHNAME
is null, the value is taken from the pathname passed with :DEFAULTS
.
For example to create the pathname of a file in the same directory as another pathname:
(make-pathname :name "bar"
:defaults (make-pathname :directory '(:absolute "tmp") :name "foo"))
Or to create a wild pathname matching the same file names but with any extension:
(make-pathname :type :wild
:defaults (make-pathname :name "foo" :type "txt"))
Or to obtain a pathname for the directory of a file:
(make-pathname :name nil
:defaults (make-pathname :directory '(:relative "a" "b" "c")
:name "foo"))
Joining two paths
Joining (or concatenating) two paths can be done with MERGE-PATHNAMES
. In
general calling (MERGE-PATHNAMES PATH1 PATH2)
returns a new pathname whose
components are taken either from PATH1
when they are not null, or from PATH2
when they are. As a special case, if the directory component of PATH1
is
relative, the directory component of the result pathname is the concatenation of
the directory components of both paths.
In other words
(merge-pathnames (make-pathname :directory '(:relative "x" "y"))
(make-pathname :directory '(:absolute "a" "b" "c")))
yields "/a/b/c/x/y/"
but
(merge-pathnames (make-pathname :directory '(:absolute "x" "y"))
(make-pathname :directory '(:absolute "a" "b" "c")))
yields "/x/y/"
.
Finding files
The DIRECTORY
function returns files matching a pathname, wild or not.
If the pathname is not wild, DIRECTORY
returns a list of one or zero element
depending on whether a file exists at this location or not.
If the pathname is wild, DIRECTORY
behaves similarly to POSIX globs. Due to
the way pathnames are structured, with the name and type being two different
components, a common error is to specify a wild name without a type. In this
case, DIRECTORY
will not return any file with an extension (since their
pathname has a non-null type). To match all files with any extension, set both
the name and the type to :WILD
.
Another interesting possibility is to only match directories. Directories are
represented by pathnames with a non-null directory component and a null name
component. Therefore to find all directories in /tmp
(top-level only):
(directory (make-pathname :directory '(:absolute "tmp" :wild)))
Note that DIRECTORY
returns truenames, i.e. pathnames representing the
canonical location of the files. An unexpected consequence is that the function
will resolve symlinks. Since the Common Lisp standard explicitely allows extra
optional arguments, some implementations have a way to disable symlink
resolving, e.g. SBCL with :RESOLVE-SYMLINKS
or CCL with :FOLLOW-LINKS
.
Resolving tildes in paths
It is commonly believed that tilde characters in paths is a universal feature. It is not. Tilde prefixes are defined in POSIX in the context of the shell (cf. POSIX 2017 2.6.1 Tilde Expansion) and are only supported in very specific locations.
To obtain the path of a file relative to the home directory of the current user,
use the USER-HOMEDIR-PATHNAME
function.
For example:
(merge-pathnames (make-pathname :directory '(:relative ".emacs.d")
:name "init" :type "el")
(user-homedir-pathname))
Joe Marshall — Get Over It (ai content)
@2025-04-25 07:00 · 77 days agoI'm tired of people complaining about all the parentheses in Lisp, so I told Gemini to vent for me. This came out pretty good.
I suppose I'm guilty of contributing to the glut of AI slop, but while the text and tone are generated, the core idea and sentiment is mine, so it isn’t pure slop.
Alright, let's drop the hand-holding. You — yes, you — the one still whimpering about the parentheses in Lisp. It's time someone told you bluntly: Get over it.
Your constant refrain about "too many parentheses" isn't some profound insight into language design; it's a confession of intellectual laziness. It screams that you encountered something different, something that didn't conform to the aesthetic you were spoon-fed, and you threw up your hands rather than engage your brain.
Are parentheses really an insurmountable cognitive barrier? Are matching pairs of symbols truly beyond your capacity to track? Let's be honest. The core issue isn't difficulty; it's unfamiliarity coupled with a stubborn refusal to adapt. You've decided what code ought to look like, and anything else is dismissed as "hard" or "ugly" without genuine effort.
Thousands upon thousands of programmers — people with brains presumably no bigger than yours — have not only managed Lisp's syntax but have thrived with it. They've built complex, powerful systems. They didn't possess some secret anti-parenthesis superpower; they simply possessed the willingness to learn and adapt. They saw the structure, the consistency, the power behind the syntax, instead of getting stuck on the superficial shape of the characters.
Your complaint isn’t about usability; it’s about preference, and a remarkably shallow one at that. It’s like refusing to read a brilliant novel because you don’t like the font. It demonstrates a lack of curiosity, an unwillingness to look past the surface.
So, here's the unvarnished truth: The parentheses aren't the problem. Your rigid thinking is. Your demand that every language cater to your pre-existing biases is.
Stop whining. Stop making excuses based on trivial syntax. Either put in the minimal mental effort required to see the structure beneath the curves, recognise the direct representation of the abstract syntax tree, and understand why it is the way it is - or don't. But don't pretend your aesthetic preference is a legitimate technical critique.
The Lisp world isn't waiting for you to overcome your parenthesis phobia. It's moving on, building things, leveraging a unique and powerful paradigm. You can either engage your intellect and join in, or you can stay on the sidelines complaining about the punctuation.
Frankly, it's time to grow up and get over the parentheses.
Marco Antoniotti — Emacs Lisp Programming with DeepSeek: A New Widget
@2025-04-24 20:52 · 77 days agoThe Emacs widget
library is useful; alas its guts are
... semi-documented and most of its inner working a bit mysterious.
I wanted a column widget where I could insert and remove a few
"line-like" widgets. The editable-list
widget does not cut it
(too many extra things: the INS and DEL buttons) and the
group
widget is too inflexible.
After too much time trying to understand all the intricacies of the
widget
library (see my rant in
my previous blog post,
which perfectly applies in this case) I asked DeepSeek to help me
out. The result, the dynamic-group
widget (after several
iterations and mistakes on part of DeepSeek) is below. It works
satisfactorlly, although it could be improved by anybody with a
better understanding of the widget
library. What is does is to
manage a colimn of line-like widgets adding and removing from the
end of the :children
list.
Check the demo-dynamic-group
for a test run.
It has been fun. Although I still want a better widget! That's why I am posting this for anybody to pitch in. Any help is welcome.
BTW. There still are some warts in the code. Can you spot them?
;;; -*- Mode: ELisp; lexical-binding: t -*- ;;; emc-dynamic-group.el ;;; ;;; `flycheck-mode' does not like the above. `flycheck-mode' is wrong. ;;; Code: (require 'widget) (require 'wid-edit) (define-widget 'dynamic-group 'default "A container widget that dynamically manages child widgets in a column." :format "%v" :value () :tag "Dynamic Group" :args nil ;; Core widget methods :create (lambda (widget) (let ((inhibit-read-only t)) (widget-put widget :from (point)) (dolist (child (reverse (widget-get widget :children))) (widget-create child)) (widget-put widget :to (point)))) :value-get (lambda (widget) (mapcar (lambda (child) (widget-apply child :value-get)) (widget-get widget :children))) :value-set (lambda (widget value) (widget-put widget :value value)) :value-delete (lambda (widget) (dolist (child (widget-get widget :children)) (widget-apply child :value-delete))) :validate (lambda (widget) (let ((children (widget-get widget :children))) (catch :invalid (dolist (child children) (when (widget-apply child :validate) (throw :invalid child))) nil))) ) (defun dynamic-group-add (widget type &rest args) "Add a new widget (of TYPE and ARGS to the WIDGET group." (let ((inhibit-read-only t)) (save-excursion (goto-char (widget-get widget :to)) (let ((child (apply 'widget-create (append (list type) args)))) (widget-put widget :children (cons child (widget-get widget :children))) (widget-put widget :to (point)) (widget-value-set widget (cons (widget-value child) (widget-value widget))))) (widget-setup))) (defun dynamic-group-remove (widget) "Remove the last widget from the WIDGET group." (when-let ((children (widget-get widget :children))) (let ((inhibit-read-only t) ;; (child (car children)) ) (save-excursion (goto-char (widget-get widget :from)) (delete-region (point) (widget-get widget :to)) (widget-put widget :children (cdr children)) (dolist (c (reverse (widget-get widget :children))) (widget-create c)) (widget-put widget :to (point)) (widget-value-set widget (mapcar 'widget-value (widget-get widget :children))) (widget-setup))))) (defun demo-dynamic-group () "Test the dynamic-group widget." (interactive) (switch-to-buffer "*Dynamic Group Demo*") (kill-all-local-variables) (let ((inhibit-read-only t)) (erase-buffer) (widget-insert "* Dynamic Group Demo\n\n") ;; Now I create the `dynamic-group'. (let ((group (widget-create 'dynamic-group))) (widget-insert "\n") ;; The rest are just two buttons testing the widget's behavior, ;; invoking`dynamic-group-add' and `dynamic-group-remove'. (widget-create 'push-button :notify (lambda (&rest _) (dynamic-group-add group 'string :format "Text: %v\n" :value (format "Item %d" (1+ (length (widget-get group :children)))))) "(+) Add Field (Click Anywhere)") (widget-insert " ") (widget-create 'push-button :notify (lambda (&rest _) (dynamic-group-remove group)) "(-) Remove Last") (widget-insert "\n")) ;; Wrap everything up using the `widget-keymap' and `widget-setup' ;; functions. (use-local-map widget-keymap) (widget-setup))) (provide 'emc-dynamic-group)
'(cheers)
Joe Marshall — Lisp Debugger Wins
@2025-04-24 18:00 · 78 days agoI'm gathering statistics from thousands of pull requests in GitHub. I wrote a little Lisp program to do that. It's taking a long time because it has to make a lot of API calls to GitHub and the calls are rate limited.
After about half an hour, I got an unexpected error. A user who made the pull request I was looking at had deleted their account. No problem. I used the Lisp debugger to walk up the stack to a convenient frame and returned NIL from that frame, causing that particular PR to be skipped. The program continued running from where it left off. I didn't have to restart from the beginning and lose a half hour of work.
The Lisp debugger for the win!
For older items, see the Planet Lisp Archives.
Last updated: 2025-07-11 14:59